A deep learning algorithm that can identify and outline (‘segment’) a non-small cell lung cancer (NSCLC) tumor on a computed tomography (CT) scan in seconds has been developed and validated by researchers. The study also found that radiation oncologists who used the algorithm in simulated clinics performed as well as physicians who did not use the algorithm, while working 65 percent faster.
Nearly half of all cases of lung cancer, the most common cancer worldwide, are treated with radiation therapy (RT). RT planning is a time-consuming, resource-intensive process that can take days to weeks to complete, and even highly trained doctors disagree on how much tissue to target with radiation. Furthermore, as cancer rates rise, a global shortage of radiation-oncology practitioners and clinics is expected to worsen.
Working under the Artificial Intelligence in Medicine Program of Mass General Brigham, Brigham and Women’s Hospital researchers and collaborators developed and validated a deep learning algorithm that can identify and outline (“segment”) a non-small cell lung cancer (NSCLC) tumor on computed tomography (CT) scan in seconds. Their study, published in the journal Lancet Digital Health, also shows that radiation oncologists who used the algorithm in simulated clinics performed as well as physicians who did not use the algorithm, while working 65 percent faster.
This study presents a novel evaluation strategy for AI models that emphasizes the importance of human-AI collaboration. This is especially necessary because in silico (computer-modeled) evaluations can give different results than clinical evaluations. Our approach can help pave the way towards clinical deployment.
Hugo Aerts
“The biggest translation gap in AI applications to medicine is the failure to study how to use AI to improve human clinicians, and vice versa,” said corresponding author Raymond Mak, MD, of the Brigham’s Department of Radiation Oncology.
“We’re studying how to make human-AI partnerships and collaborations that result in better outcomes for patients. The benefits of this approach for patients include greater consistency in segmenting tumors and accelerated times to treatment. The clinician benefits include a reduction in mundane but difficult computer work, which can reduce burnout and increase the time they can spend with patients.”
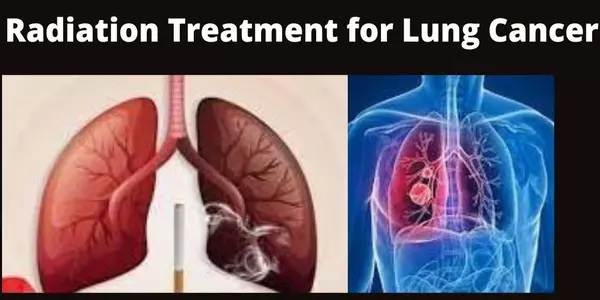
The researchers used CT images from 787 patients to train their model to distinguish tumors from other tissues. They tested the algorithm’s performance using scans from over 1,300 patients from increasingly external datasets. Developing and validating the algorithm involved close collaboration between data scientists and radiation oncologists. For example, when the researchers observed that the algorithm was incorrectly segmenting CT scans involving the lymph nodes, they retrained the model with more of these scans to improve its performance.
Finally, the researchers had eight radiation oncologists perform segmentation tasks as well as rate and edit segmentations created by another expert physician or the algorithm (they were not told which). The performance of human-AI collaborations and human-produced (de novo) segmentations did not differ significantly.
Intriguingly, when editing an AI-produced segmentation versus a manually produced one, physicians worked 65 percent faster and with 32 percent less variation, even though they had no idea which one they were editing. In this blinded study, they also rated the quality of AI-drawn segmentations higher than the quality of human expert-drawn segmentations.
In the future, the researchers intend to combine this work with previously developed AI models that can identify “organs at risk” of receiving unwanted radiation during cancer treatment (such as the heart) and thus exclude them from radiotherapy. They are continuing to research how physicians interact with AI in order to ensure that AI partnerships benefit clinical practice rather than harm it, and they are developing a second, independent segmentation algorithm that can verify both human and AI-drawn segmentations.
“This study presents a novel evaluation strategy for AI models that emphasizes the importance of human-AI collaboration,” said co-author Hugo Aerts, Ph.D., of the Department of Radiation Oncology. “This is especially necessary because in silico (computer-modeled) evaluations can give different results than clinical evaluations. Our approach can help pave the way towards clinical deployment.”