Physicists collaborated with computer specialists to create an event-based architecture based on photonic processors. This, like the brain, allows for ongoing adaptation of the connections within the neural network.
Scientists from the University of Münster (Germany), lead by physicists Prof. Wolfram Pernice, Prof. Martin Salinga, and computer scientist Prof. Benjamin Risse, created an event-based architecture employing photonic processors. This, like the brain, allows for ongoing adaptation of the connections within the neural network.
Modern computer models, such as those used in complicated, powerful AI applications, test the limits of classical digital computer operations. New computing architectures that mimic the operating principles of biological neural networks hold the promise of quicker, more energy-efficient data processing.
Our goal is to create an optical computing architecture that will allow us to compute AI applications quickly and efficiently in the long run.
Frank Brückerhoff-Plückelmann
A group of academics has now created an event-based architecture that use photonic processors to transfer and analyze data via light. This, like the brain, allows for ongoing adaptation of the connections within the neural network. Changeable connections serve as the foundation for learning processes.
A team from the University of Münster’s Collaborative Research Centre 1459 (“Intelligent Matter”), led by physicists Prof. Wolfram Pernice and Prof. Martin Salinga, and computer specialist Prof. Benjamin Risse, collaborated with researchers from the Universities of Exeter and Oxford in the United Kingdom for the study. The research was reported in the journal “Science Advances.”
What is needed for a neural network in machine learning are artificial neurons which are activated by external excitatory signals, and which have connections to other neurons. The connections between these artificial neurons are called synapses – just like the biological original.
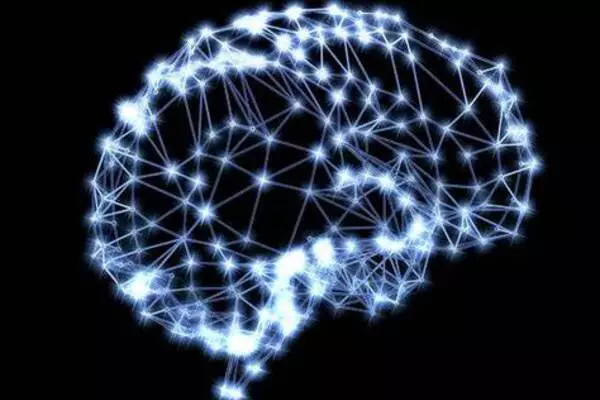
The Münster team used a network of nearly 8,400 optical neurons made of waveguide-coupled phase-change material for their study, and the team demonstrated that the connection between two of these neurons can indeed become stronger or weaker (synaptic plasticity), and that new connections can be formed or existing ones eliminated (structural plasticity).
In contrast to past similar studies, the synapses were coded as a result of the attributes of the optical pulses – that is, as a result of the respective wavelength and strength of the optical pulse. This enabled the integration of thousands of neurons on a single chip and optically connecting them.
Light-based computers have substantially better bandwidth than typical electronic processors, allowing for the execution of complicated computational tasks while using less energy. This innovative technique is based on fundamental research. “Our goal is to create an optical computing architecture that will allow us to compute AI applications quickly and efficiently in the long run,” says Frank Brückerhoff-Plückelmann, one of the study’s primary authors.
Methodology: The non-volatile phase-change material can transition between amorphous and crystalline structures with a highly organized atomic lattice. This feature enables persistent data storage even in the absence of an electrical supply. The neural network’s performance was evaluated by using an evolutionary approach to teach it to discriminate between German and English texts. The number of vowels in the text served as the recognition parameter.