Machine learning algorithms were used to evaluate X-ray diffraction data and forecast material crystal structures. This is especially important when traditional approaches are time-consuming or difficult. In groundbreaking work that could drive the discovery of new mathematical conclusions, researchers employed machine learning to discover the qualities of atomic fragments of geometry.
For the first time, mathematicians from the University of Nottingham and Imperial College London employed Machine Learning to expand and speed work on identifying ‘atomic shapes’ that compose the basic elements of geometry in higher dimensions. Their findings were reported in the journal Nature Communications.
This is where Artificial Intelligence could really revolutionise Mathematics as we have shown that machine learning is a powerful tool for spotting patterns in complex domains like algebra and geometry.
Professor Tom Coates
Several years ago, the research team began work on developing a Periodic Table of Shapes. The atomic fragments are known as Fano variants. Each form is assigned a sequence of numbers known as quantum periods, which results in a ‘barcode’ or ‘fingerprint’ that describes the shape. Their current achievement employs a novel machine learning technology to rapidly sift through these barcodes, detecting forms and their attributes such as each shape’s dimension.
Alexander Kasprzyk is an Associate Professor in Geometry in the School of Mathematical Sciences at the University of Nottingham and was one of the authors of the paper. He explains: “For mathematicians, the key step is working out what the pattern is in a given problem. This can be very difficult, and some mathematical theories can take years to discover.”
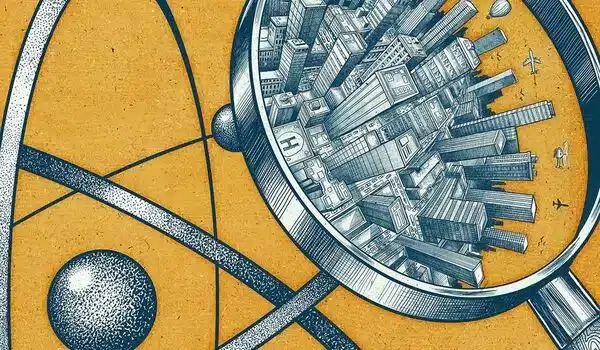
Professor Tom Coates from the Department of Mathematics at Imperial College London and co-author on the paper said, “This is where Artificial Intelligence could really revolutionize Mathematics as we have shown that machine learning is a powerful tool for spotting patterns in complex domains like algebra and geometry.”
It is computationally demanding to solve sophisticated quantum mechanical equations for big systems. Machine learning models, such as neural networks, have been trained to anticipate quantum mechanical features and behaviors, thereby assisting in the comprehension of atomic and molecule structures.
Machine learning has been used to analyze molecular dynamics simulations, allowing researchers to gain insight into the long-term behavior of atoms and molecules. This includes forecasting atom trajectories and comprehending material thermodynamic characteristics.
“We’re really excited about the fact that machine learning can be used in Pure Mathematics,” says Sara Veneziale, co-author and PhD student on the team. This will hasten new discoveries in the sector.”