The number of calories you need to consume to lose weight is determined by a variety of factors such as your current weight, height, age, gender, activity level, and weight loss goals. However, as a general rule, you must create a calorie deficit by consuming fewer calories than your body requires to maintain its current weight.
Keeping track of everything you eat and drink in a day is a time-consuming task that becomes difficult to maintain over time. Unfortunately, diligent tracking is required for successful weight loss; however, a new study published in Obesity finds that perfect tracking is not required for significant weight loss.
For six months, researchers from UConn, the University of Florida, and the University of Pennsylvania tracked 153 weight loss programme participants who self-reported their food intake using a commercial digital weight loss programme. The researchers wanted to know what the best diet tracking thresholds were for predicting 3%, 5%, and 10% weight loss after six months.
“We partnered with WeightWatchers because they were about to launch a new Personal Points programme and wanted empirical data through our clinical trial,” says co-author and Department of Allied Health Sciences Professor Sherry Pagoto.
One interesting aspect of this data is that, in the literature, researchers frequently examine whether there is a correlation between tracking and overall weight loss outcomes. Ran applied data science to the data and discovered there is more to the story.
Sherry Pagoto
According to Pagoto, the new programme takes a personalised approach to point assignment, including a list of zero-point foods to eliminate the need to calculate calories for everything.
“Dietary tracking is an essential component of all weight loss interventions, and it is the best predictor of outcomes. This programme alleviates the burden of that task by allowing zero-point foods that do not require tracking.”
Researchers and developers are looking for ways to make the tracking process less onerous, because, as Pagoto points out, many programmes make users feel as if they have to count calories for the rest of their lives: “That’s just not sustainable.” Is it necessary for users to track everything every day or not?”
Assistant Professor in the Department of Allied Health Sciences Ran Xu wanted to see if there was a way to predict outcomes based on how much diet tracking participants did with six months of data. Ran Xu and Allied Health Sciences Ph.D. student Richard Bannor examined the data to see if there were data science patterns associated with weight loss success. They discovered how many days people needed to track their food to achieve clinically significant weight loss using a method known as receiver operating characteristics (ROC) curve analysis.
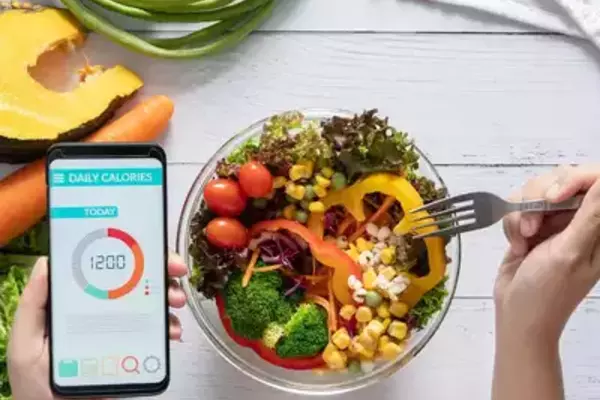
“It turns out that you don’t have to track 100% of the time to be successful,” Xu says. “In this trial, we discovered that people only need to track around 30% of the time to lose more than 3% of their body weight, 40% of the time to lose more than 5% of their body weight, and nearly 70% of the time to lose more than 10% of their body weight.” The key point here is that you don’t have to track every day in order to lose clinically significant weight.”
This is encouraging because, according to Pagoto, the goal for a six-month weight loss programme is typically 5% to 10%, a range where clinical trials have shown health benefits.
“A lot of people believe they need to lose 50 pounds to get healthier,” says Pagoto. “However, we start to see changes in things like blood pressure, lipids, cardiovascular disease risk, and diabetes risk when people lose about 5-to-10% of their weight. This is achievable if participants lose one to two pounds per week, which is considered a healthy rate of weight loss.”
Xu then examined diet tracking trajectories over the course of the program’s six months.
The scientists discovered three distinct trajectories. One group, known as high trackers or super users, tracked their food on most days of the week for six months and lost 10% of their body weight on average.
However, many participants belonged to a second group that began tracking regularly before gradually decreasing to about one day per week by the four-month mark. They still lost 5% of their body weight.
A third group, known as the low trackers, began tracking only three days per week and dropped to zero after three months, where they remained for the duration of the intervention. This group lost only 2% of their body weight on average.
“One interesting aspect of this data is that, in the literature, researchers frequently examine whether there is a correlation between tracking and overall weight loss outcomes. Ran applied data science to the data and discovered there is more to the story,” Pagoto says. “We’re now seeing different tracking patterns. This will help us determine when to provide additional assistance and who will require it the most.”
The patterns could help shape future programmes that are tailored to improve user tracking based on which group they belong to. Future research will delve deeper into these patterns to discover why they occur and, hopefully, develop interventions to improve outcomes.
“For me, what’s exciting about these digital programmes is that we have a digital footprint of participant behaviour,” Xu says. “We can get into the weeds of what people do during these programmes.” The data can be used to inform precision medicine approaches, in which we can use data science to identify patterns of behaviour and design a targeted approach.”
Digitally delivered health programmes provide researchers with a plethora of data they did not previously have, which can yield new insights, but this science necessitates a multidisciplinary approach.
“Before, it felt like we were flying in the dark or relying on anecdotes or self-reported measures, but now that we have so much user data, it’s different.” To make sense of all this data, we need data science. This is where team science comes into play, because clinical and data scientists approach the problem from very different perspectives, but when we work together, we can produce insights that neither of us could produce alone. “This has to be the future of this work,” Pagoto says.
“From a data science standpoint, machine learning is exciting,” says Xu, “but if we only have machine learning, we only know what people do, but we don’t know why or what to do with this information.” This is where clinical scientists like Sherry come in to help us make sense of the data. That is why team science is so critical.”