Quail are small, ground-dwelling birds that belong to the family Phasianidae, which also includes chickens, pheasants, and turkeys. There are several species of quail, including the northern bobwhite, California quail, and mountain quail. Quail are known for their plump bodies, short wings, and short, rounded tails. They are typically found in open woodlands, grasslands, and scrublands and feed on seeds, berries, and insects. Some species of quail are hunted for sport, while others are raised for their eggs and meat.
When states want to assess quail populations, the process can be difficult, time-consuming, and costly. It entails spending hours in the field listening for calls. Or leaving a recording device in the field to capture what sounds are made, only to spend hours later listening to that audio. Then, repeat the process until there is enough information to begin making population estimates.
However, a new model developed by researchers at the University of Georgia aims to streamline this process. The process, which uses artificial intelligence to analyze terabytes of quail call recordings, allows wildlife managers to gather the data they need in a matter of minutes.
The model is very accurate, picking up between 80% and 100% of all calls even in the noisiest recordings. So, you could take a recording, put it through our model and it will tell you how many quail calls that the recorder heard.
James Martin
“The model is very accurate, picking up between 80% and 100% of all calls even in the noisiest recordings. So, you could take a recording, put it through our model and it will tell you how many quail calls that the recorder heard,” said James Martin, an associate professor at the UGA Warnell School of Forestry and Natural Resources who has been working on the project, in collaboration with the Georgia Department of Natural Resources, for about five years.
“This new model allows you to analyze terabytes of data in seconds, and what that will allow us to do is scale up monitoring, so you can literally put hundreds of these devices out and cover a lot more area with a lot less effort than in the past.”
The software represents about five years of work by Martin, postdoctoral researcher Victoria Nolan, and numerous key contributors who collaborated with a code writer to create the model. It’s also part of a larger shift in the field of wildlife research, where computer algorithms are now assisting with work that used to take humans thousands of hours to complete.
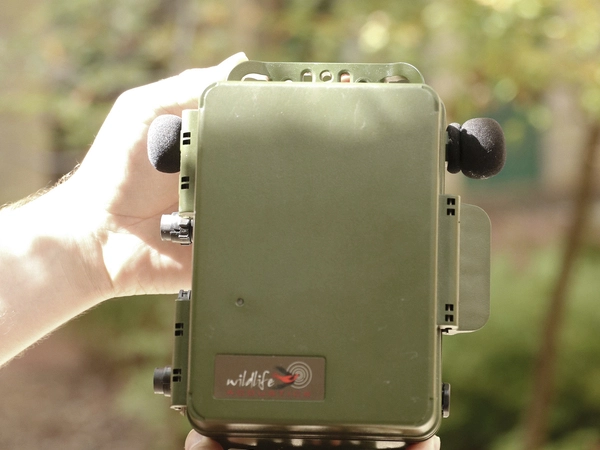
Computers are becoming smarter at recognizing specific noises or traits in photos and sound recordings, for example. For researchers like Martin, this means that hours previously spent on tasks like listening to audio or inspecting game camera images can now be completed by a computer, freeing up valuable time to focus on other aspects of a project.
The new tool could also be useful for state and federal agencies seeking information on their quail populations but with limited funds to spend on any one project. “So, I think this is something that states might jump on in terms of replacing their current monitoring with acoustic recording devices,” Martin added.
The software’s success was recently documented by the Journal of Remote Sensing in Ecology and Conservation.
Martin claims that as the software is used more and exposed to sounds from new geographic areas, it becomes “smarter.” Quail already have several different types of calls. However, when the software is exposed to a variety of non-quail sounds, he claims that it becomes better at distinguishing the correct calls from the ambient noises of the grasses and trees around them.
The software will become more discriminating over time. “That’s why you have to keep giving it training data, and when you move geographies, you run into new sounds that you didn’t train the model for,” he explained. “It’s all about adaptation.”