Automation, or the replacement of humans in the workplace by machines, is not a new concept, but digital technology has greatly expanded the capabilities of these machines. Machines in factories, for example, produce cars incessantly, precisely, and quickly, and these cars are increasingly capable of driving themselves, posing urgent ethical challenges. Artificial intelligence (AI) enables these machines to perform complex tasks with little or no supervision.
A robot is one such machine that senses, thinks, and acts autonomously when it does so without external control. The appearance of these machines is sometimes entirely determined by function, but when that function is to interact with humans, they may be given anthropomorphic form. Humanoid robots are designed to look and act like humans, and they usually have some way of communicating with them.
Researchers describe how a robot can be taught to navigate a maze by electrically stimulating a culture of brain nerve cells linked to the machine. These nerve cells were created from living cells and served as a physical reservoir for the computer to use in order to generate coherent signals. These findings suggest that by sending disturbance signals to an embodied system, goal-directed behavior can be generated without any additional learning.
Researchers describe how a robot can be taught to navigate a maze by electrically stimulating a culture of brain nerve cells connected to the machine. These nerve cells, or neurons, were grown from living cells and served as the computer’s physical reservoir for constructing coherent signals.
Hirokazu Takahashi
Can robots be taught intelligence? Advances in physical reservoir computing, a technology that interprets brain signals, could aid in the development of artificial intelligence machines that think like humans.
Researchers from the University of Tokyo describe how a robot can be taught to navigate a maze by electrically stimulating a culture of brain nerve cells connected to the machine in AIP Publishing’s Applied Physics Letters. These nerve cells, or neurons, were grown from living cells and served as the computer’s physical reservoir for constructing coherent signals.
The signals are thought to be homeostatic signals, informing the robot that its internal environment was kept within a certain range and serving as a baseline as it moved freely through the maze.
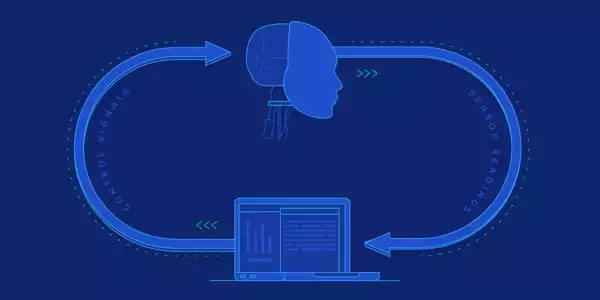
An electric impulse disrupted the neurons in the cell culture whenever the robot veered in the wrong direction or faced the wrong way. Throughout the trials, the robot was fed homeostatic signals that were interrupted by disturbance signals until it successfully completed the maze task.
These findings suggest that by sending disturbance signals to an embodied system, goal-directed behavior can be generated without any additional learning. Because the robot couldn’t see its surroundings or gather other sensory information, it was completely reliant on electrical trial-and-error impulses.
“Our experiments inspired me to hypothesize that intelligence in a living system emerges from a mechanism extracting a coherent output from a disorganized or chaotic state,” said co-author and associate professor of mechano-informatics Hirokazu Takahashi.
Using this principle, the researchers demonstrate that intelligent task-solving abilities can be produced by extracting chaotic neuronal signals and delivering homeostatic or disturbance signals using physical reservoir computers. As a result, the computer builds a reservoir that understands how to complete the task.
“A brain of [an] elementary school child is unable to solve mathematical problems in a college admission exam, possibly because the dynamics of the brain or their ‘physical reservoir computer’ is insufficiently rich,” Takahashi explained. “Task-solving ability is determined by the network’s ability to generate a diverse repertoire of spatiotemporal patterns.”
The researchers believe that using physical reservoir computing in this context will help them better understand the brain’s mechanisms and may lead to the development of a novel neuromorphic computer.
Robots in the classroom, for example, can serve a variety of purposes. Some are objects of study for students to practice programming, others are tools to assist a teacher, some can be learning companions, and others may be autonomous teachers that provide some unit of instruction in its entirety or in part.
The future of artificial intelligence (AI) is both uncertain and a hot topic of debate among the world’s technology visionaries. On one side of the debate, AI has been portrayed as a potential threat to humanity, while on the other, it has been portrayed as a beneficial extension of our existence.