Predicting city traffic can be challenging as it can be influenced by a wide range of factors such as time of day, day of the week, weather conditions, road construction or accidents, and major events in the city.
A new machine learning model can forecast traffic activity in various city zones. A Complexity Science Hub researcher did this by using data from a major car-sharing company in Italy as a proxy for overall city traffic. Understanding how different urban zones interact, for example, can help avoid traffic jams and enable policymakers to respond in a targeted manner, such as local expansion of public transportation.
Understanding people’s mobility patterns is critical for improving urban traffic flow. “As urban populations grow, this knowledge can help policymakers design and implement effective transportation policies and inclusive urban planning,” says Complexity Science Hub’s Simone Daniotti.
For example, if the model reveals a nontrivial connection between two zones, i.e., that people commute from one zone to another for various reasons, services that compensate for this interaction could be provided. If, on the other hand, the model indicates that there is little activity in a specific location, policymakers can use that information to invest in structures to change that.
Of course, making predictions is important, but you can make very accurate predictions, and if you don’t interpret the results correctly, you sometimes risk drawing very wrong conclusions. Inspecting and understanding the model helps us, as well as policymakers, avoid drawing incorrect conclusions.
Simone Daniotti
Model Also for Other Cities Like Vienna
A major car-sharing company provided the data for this study, which included the location of all cars in their fleet in four Italian cities (Rome, Turin, Milan, and Florence) in 2017. The information was gathered by repeatedly querying the service provider’s web APIs, noting the parking location of each vehicle as well as the start and end timestamps. “With this information, we can determine the origin and destination of each trip,” Daniotti explains.
Daniotti used this as a proxy for all city traffic and developed a model that not only allows accurate spatiotemporal forecasting in various urban areas, but also accurate anomaly detection. Anomalies such as strikes and inclement weather, both of which are related to traffic. The model could also make predictions about traffic patterns for other cities such as Vienna. “However, this would require appropriate data,” Daniotti points out.
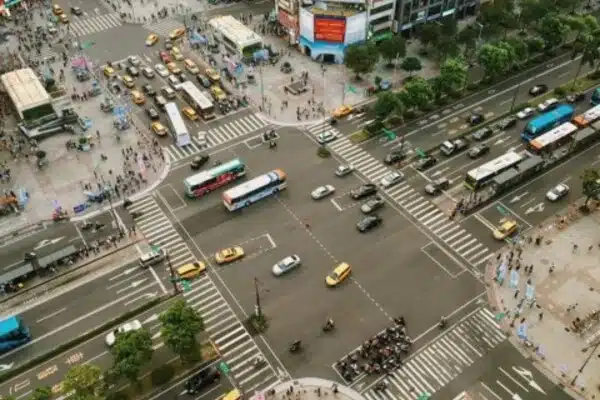
Outperforming Other Models
While there are many models that predict traffic behavior in cities, “The vast majority of prediction models based on aggregated data are unintelligible. Even though some model structure connects two zones, this cannot be interpreted as an interaction “Daniotti elaborates. This limits comprehension of the underlying mechanisms that govern citizens’ daily lives. The new model is fully interpretable because only a small number of constraints are considered and all parameters represent actual interactions.
But What Is Prediction Without Interpretation?
“Of course, making predictions is important,” Daniotti says, “but you can make very accurate predictions, and if you don’t interpret the results correctly, you sometimes risk drawing very wrong conclusions.”
It is difficult to control for events where the model did not produce the expected results unless you know why the model produced that result. “Inspecting and understanding the model helps us, as well as policymakers, avoid drawing incorrect conclusions,” Daniotti says.
It is important to note that predicting city traffic can be a complex task, and there is always some level of uncertainty involved. However, by using historical data, machine learning models, and real-time data, it is possible to make more accurate predictions about traffic patterns and help to improve traffic management in cities.