The study investigates the functions of the nervous system of the fruit fly in food-seeking; the findings are useful for the development and control of artificial intelligence. Insects can cope with problems in their environments by changing their behavior based on previous experiences. To form a distributed memory, their nervous systems use fast, parallel processing with brief nerve impulses.
Insects such as dragonflies, honeybees, and fruit flies are adept at navigating their surroundings. As a result, their visual processing abilities are very impressive. They can change direction in the blink of an eye to catch prey or avoid collisions. Because self-driving cars will need to perform similar tasks, insects’ visual processing abilities are of particular interest. Insects are very good at extracting information about their surroundings from low-resolution data.
The nervous systems of insects were studied by zoologists at the University of Cologne in order to investigate principles of biological brain computation and potential implications for machine learning and artificial intelligence. They studied how insects learn to associate sensory information in their environment with a food reward, and how they can recall this information later in order to solve complex tasks like food search. The findings imply that the transformation of sensory information into memories in the brain may inspire future machine learning and artificial intelligence applications for complex task solving. The findings were published in the journal PNAS.
We initially trained our fly brain model in the same way that insects are trained in experiments.’ In the simulation, we presented a specific scent along with a reward and a second scent without a reward. After only a few scent presentations, the model quickly learns a robust representation of the rewarded scent and is then able to find the source of this scent in a spatially complex and temporally dynamic environment.
Dr. Hannes Rapp
Living organisms have extraordinary abilities to deal with problems posed by complex and dynamic environments. They can generalize their experiences and quickly adapt their behavior when the environment changes. The zoologists looked into how the nervous system of a fruit fly controls its behavior when looking for food. They simulated and analyzed the computations in the fruit fly’s nervous system in response to scents emitted by the food source using a computer model.
‘We initially trained our fly brain model in the same way that insects are trained in experiments.’ In the simulation, we presented a specific scent along with a reward and a second scent without a reward. After only a few scent presentations, the model quickly learns a robust representation of the rewarded scent and is then able to find the source of this scent in a spatially complex and temporally dynamic environment,’ said computer scientist Dr Hannes Rapp, who developed the model as part of his doctoral thesis at the University of Copenhagen’s Institute of Zoology.
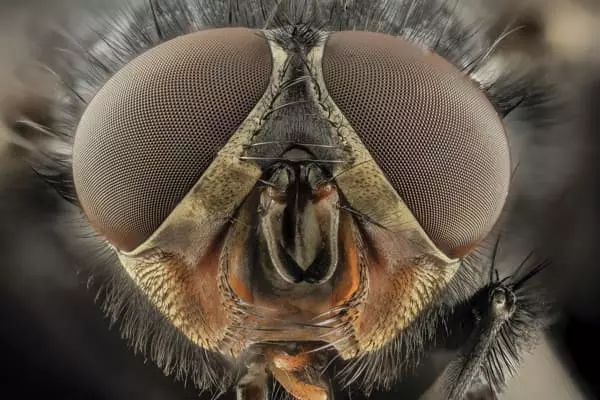
The model developed is thus capable of generalizing from memory and applying what it has previously learned in a completely new and complex odour molecule landscape, despite the fact that learning required only a small database of training samples. ‘For our model, we exploit the special properties of biological information processing in nervous systems,’ said senior author Professor Dr Martin Nawrot. ‘These include the rapid and parallel processing of sensory stimuli via brief nerve impulses, as well as the formation of a distributed memory via the simultaneous modification of many synaptic contacts during the learning process.’ The theoretical principles underlying this model can also be applied to artificial intelligence and self-driving cars. They enable an artificial agent to learn much more efficiently and to apply what it has learned in a changing environment.
The researchers studied how fruit flies learn to find food in their surroundings and developed a computational model that mimics the process. Typically, researchers train flies by presenting one scent with a reward and another without a reward. The fly quickly learns to associate certain scents with rewards, and they can use this knowledge to find food in more complex environments in the future.
The researchers examined the computational processing involved in the process and applied it to a computational model that mimics fruit flies’ biological information processing. Biological computation is distinguished by its use of fast and parallel processing with input from brief nerve pulses. Furthermore, throughout the learning process, it constantly changes and modifies contacts between neurons, resulting in a distributed memory. This enables a machine learning model, such as AI or an autonomous system, to learn much more efficiently and apply what it has learned in a changing environment, despite having a small database of training samples.