An international team of researchers is developing a brain-interface system that combines soft scalp electronics and virtual reality. A new wearable brain-machine interface (BMI) system could help people with motor dysfunction or paralysis, as well as those suffering from locked-in syndrome, which occurs when a person is fully conscious but unable to move or communicate.
A multi-institutional, international team of researchers led by Woon-Hong Yeo’s lab at the Georgia Institute of Technology developed a BMI system that allows the user to imagine an action and wirelessly control a wheelchair or robotic arm.
The team, which included researchers from the University of Kent in the United Kingdom and Yonsei University in the Republic of Korea, describes the new motor imagery-based BMI system in the journal Advanced Science, which was published this month.
Researchers have developed a wearable brain-machine interface (BMI) system that they believe could improve the quality of life for people with motor dysfunction or paralysis.
“The main advantage of this system to the user, compared to what is currently available, is that it is soft and comfortable to wear, with no wires,” said Yeo, an associate professor at the George W. Woodruff School of Mechanical Engineering.
BMI systems are a type of rehabilitation technology that analyzes a person’s brain signals and converts them into commands, converting intentions into actions. ElectroEncephaloGraphy, or EEG, is the most common non-invasive method for acquiring those signals. It typically requires a cumbersome electrode skull cap and a tangled web of wires.
These devices are generally inconvenient and uncomfortable to use, rely heavily on gels and pastes to help maintain skin contact, and require lengthy set-up times. In addition, the devices frequently suffer from poor signal acquisition due to material degradation or motion artifacts – the ancillary “noise” caused by things like teeth grinding or eye blinking. This noise appears in brain data and must be removed.
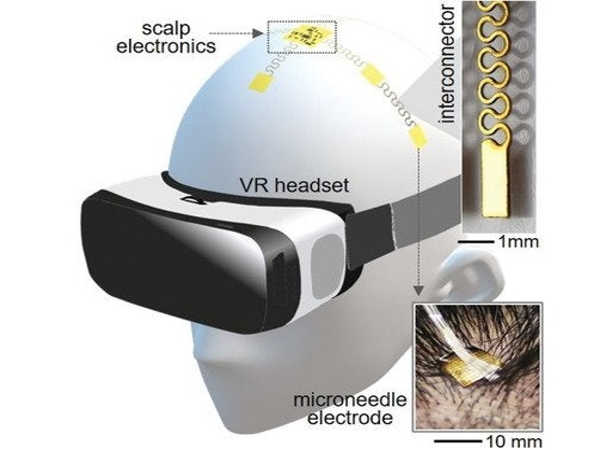
Yeo’s portable EEG system, which combines imperceptible microneedle electrodes with soft wireless circuits, improves signal acquisition. Accurately measuring those brain signals is critical for determining what actions a user wants to take, so the team used a powerful machine learning algorithm and a virtual reality component to address that challenge.
The new system has been tested on four human subjects, but it has not yet been studied on disabled people. “This is just the beginning,” said Yeo, Director of Georgia Tech’s Center for Human-Centric Interfaces and Engineering in the Institute for Electronics and Nanotechnology and a member of the Petit Institute for Bioengineering and Bioscience.
New Paradigm
In a 2019 study published in Nature Machine Intelligence, Yeo’s team first described a soft, wearable EEG brain-machine interface. Musa Mahmood, the work’s lead author, was also the lead author of the team’s new research paper.
“This new brain-machine interface uses an entirely different paradigm, involving imagined motor actions, such as grasping with either hand, which frees the subject from having to look at too many stimuli,” Mahmood, a Ph. D. student in Yeo’s lab, explained.
Users demonstrated accurate control of virtual reality exercises using their thoughts – their motor imagery – in the 2021 study. The visual cues improve the process of gathering information for both the user and the researchers.
“The virtual prompts have proven to be extremely useful,” Yeo said. “They increase user engagement and accuracy while also speeding up the process. Furthermore, we were able to capture continuous, high-quality motor imagery activity.”
Using what they’ve learned from the previous two studies, Mahmood says that future work on the system will focus on optimizing electrode placement and more advanced integration of stimulus-based EEG.