Following the 2016 U.S. election, I set out to create a solution that would combat the pandemic of fake news on the internet. My first concept was simple: create a semi-automated fact-checking program that could detect any false or doubtful claim and recommend the highest-quality contextual facts for it.
Our concept was simple, if not utopian: if technology could encourage people to seek truth, facts, figures, and data in order to make judgments, we could create an online dialogue based on reason and logic rather than exaggeration. Factmata has achieved some success after five years of hard labor. However, in order, this area genuinely develop a number of obstacles must addressed, ranging from economic to technical.
We immediately found that automated fact-checking is a difficult research topic to solve. The first hurdle was determining which facts we needed to verify. Then there was the question of how we might create and maintain up-to-date databases of facts that would allow us to evaluate the accuracy of various assertions. The widely used Wikidata knowledge base, for example, seemed an obvious choice, but it updated too slowly to verify claims about fast-changing events.
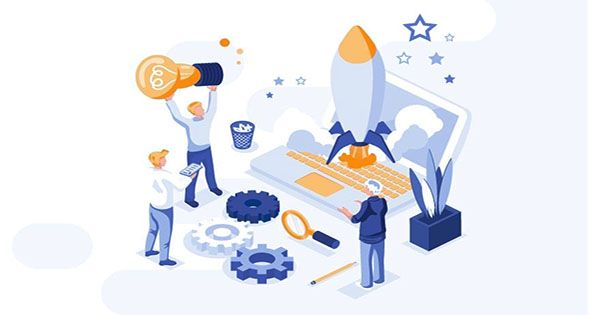
We also learned that being a for-profit fact-checking firm posed challenges. Most journalistic and fact-checking organizations are non-profits, and social media companies want to cooperate with them to avoid bias charges. Beyond these considerations, establishing a company that can assess what is “excellent” is intrinsically difficult and intricate. Definitions are a never-ending source of contention. What labeled “fake news” was frequently excessive hyperpartisanship, what labeled “misinformation” was really opposing viewpoints.
From a commercial viewpoint, we felt that recognizing what was “bad” (toxic, vulgar, threatening, or hateful) was a far easier path. We chose to detect “gray area” hazardous text, which is stuff that a platform is unsure should eliminate but requires more contexts. To do this, we created an API that assesses the harmfulness of comments, postings, and news items based on their hyperpartisanship, controversiality, objectivity, hatefulness, and 15 other indicators.
We felt there was benefit in keeping track of all the assertions regarding significant corporate issues that were made online. As a result, in addition to our API, we developed a SaaS platform that tracks rumors and “narratives” in any issue, whether it is about a brand’s products, government policy, or COVID-19 vaccinations. It is understandable if this appears to be a difficult task. One of the most important lessons we learnt was how little a $1 million initial investment gets you in this market. Data training around verified hate speech and misleading claims is not a simple labeling exercise; it needs subject-matter knowledge and meticulous thought, neither of which is inexpensive.
In reality, developing the tools we required — which included numerous browser extensions, website demos, a data-labeling platform, social news commenting platform, and live real-time dashboards of our AI’s output — felt like launching many new businesses at once.
To make matters even more complicated, determining product-market fit was a difficult task. Factmata has switched its focus after many years of development to brand safety and reputation.
We offer our technology to online advertising platforms that want to clean up their ad inventory, companies that want to manage and optimize their reputation, and smaller platforms that need content moderation.
We have taken a long time to get to this point, but in the last year, we have finally seen many clients sign up for monthly trials and contracts, and we are on track to hit $1 million in recurring income by mid-2022.