To date, one of the most prevalent applications of artificial intelligence has been the employment of computers trained with previous data to predict future outcomes. However, fame may not always imply success: As others have pointed out (and as we have seen), predictive AI leaves out a lot of the nuance, context, and cause-and-effect reasoning that goes into an outcome.
As a result, predictive AI’s “logical” answers can sometimes be disastrous. CausaLens, a firm that believes it can tackle this challenge, has developed causal inference technology — marketed as a no-code tool that does not require a data scientist to use to introduce more complexity, reasoning, and cause-and-effect sensibility into an AI-based system.
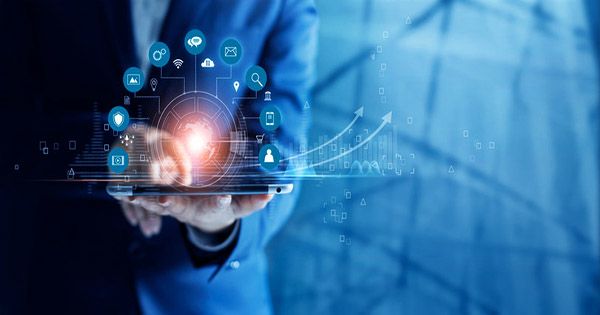
The goal of CausaLens, according to CEO and co-founder Darko Matovski, is for AI to “begin to perceive the world like humans do.” After experiencing early success with its method, the business is announcing $45 million in fundraising today, having grown revenues 500 percent since coming out of stealth a year ago. This touted as the rounds “first close,” means it is still open and might expand in size. Dorilton Ventures and Molten Ventures (which rebranded from Draper Esprit) led the round, which also included former investors Generation Ventures and IQ Capital, as well as new backer GP Bullhound. The financing puts London-based causaLens at roughly $250 million, according to sources.
Customers and partners of CausaLens include companies in the healthcare, financial services, and government sectors, among others, where the company’s technology utilized not only for AI-based decision making but also to add additional cause-and-effect depth to results. The Mayo Clinic, one of the startup’s partners, has been utilizing causaLens to identify cancer biomarkers, which is an excellent illustration of how this works.
“Human bodies are complex systems,” Darko Matovski, the startup’s CEO and founder, said in an interview. “Using basic AI paradigms, you may identify any pattern you want, any correlations of any kind, and you’re not getting anywhere.” “However, by using cause and effect techniques to grasp the mechanics of how different bodies work, you can gain a better understanding of how one portion affects another.”
Given all of the variables involved, it the type of big data problem that would be practically difficult for a human, or even a team of humans, to solve, but is trivial for a computer to solve. While this research is not a cure for cancer, it is a huge step toward considering different treatments suited to the many variables involved. CausaLens’ technology has also been used in healthcare in a less clinical fashion. A public health agency from one of the world’s largest economies (causaLens cannot reveal one) used causal AI engine to figure out some adults have resisting COVID-19 vaccinations, so the agency could devise better strategies to get them on board (plural “strategies” is the operative detail here.
The whole point is that it is a complicated issue involving a variety of reasons depending on the individuals in question). Other customers in industries such as financial services have used causaLens to feed automated decision-making algorithms in areas such as loan evaluations, where earlier AI systems introduced bias into their choices by relying solely on historical data.
Hedge funds, on the other hand, employ causaLens better understand a market trend can develop and use that information to inform their investment plans. Intriguingly, in the world of autonomous mobility, a new group of clients may emerge. This is one area where a lack of human reasoning has stymied advancement in the subject.