A new context-aware robot system is ready for deployment on the factory floor. According to a recent study, the system is more efficient because it can recognize coworkers and their body shapes, as well as predict their movements.
Working safely entails more than just processes; it also entails understanding the work environment and circumstances, as well as predicting what other people will do next. A new system gives robots this level of context awareness, allowing them to work more efficiently and without unnecessary interruptions on assembly lines alongside humans.
Robots must not only be integrated into processes for safe collaboration; they must also understand the production environment and be able to predict what humans will do next. Last week, researchers at the KTH Royal Institute of Technology announced the development of a system that provides context awareness, allowing robots to work more safely and efficiently alongside humans.
Study shows the system is more efficient because it can recognize co-workers and their body shapes, and even predict their movements.
According to Hongyi Liu, a researcher at KTH Royal Institute of Technology, the human-robot collaboration system can identify each worker it works with, as well as the person’s skeleton model, which is an abstract of body volume, instead of only judging distance between itself and its human co-workers. The context-aware robot system can recognize the worker’s pose and even predict the next pose using this information. These abilities provide a context for the robot to be aware of while interacting.
According to Liu, KTH’s system employs artificial intelligence, which requires less computational power and smaller datasets than traditional machine learning methods. It instead employs a type of machine learning known as transfer learning, which repurposes knowledge gained through training before being adapted into an operational model.
According to Liu, the system employs artificial intelligence, which necessitates less computational power and smaller datasets than traditional machine learning methods. It instead employs a type of machine learning known as transfer learning, which reuses knowledge gained through training before being adapted into an operational model.
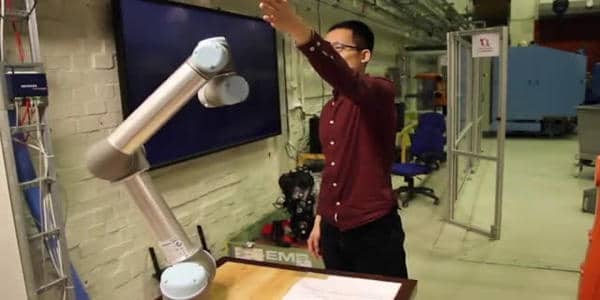
The study was co-authored by KTH Professor Lihui Wang and published in the recent issue of Robotics and Computer-Integrated Manufacturing. According to Liu, the technology is far ahead of today’s International Organization for Standards (ISO) requirements for collaborative robot safety, so implementation would necessitate industrial action. However, he claims that context-awareness is more efficient than the one-dimensional interaction that workers currently have with robots.
“When a human approaches a robot, it slows down, and if he or she gets close enough, it stops, according to ISO standards and technical specifications. It resumes if the person moves away. That’s a very low level of contextual awareness “he claims.
“It jeopardizes productivity. Production is slowed, and humans are unable to work closely with robots.” Liu compares the context-aware robot system to a self-driving car that notices how long a stoplight has been red and anticipates when it will be green again. Rather than braking or downshifting, it begins to adjust its speed by cruising toward the intersection, sparing the brakes and transmission from further wear.
Experiments with the system revealed that context allows a robot to operate more safely and efficiently without slowing down production. During one of the system’s tests, a robot arm’s path was unexpectedly blocked by someone’s hand. Instead of stopping, the robot adjusted; it predicted the hand’s future trajectory, and the arm moved around the hand. “This is safety not only from a technical standpoint in avoiding collisions, but also from recognizing the context of the assembly line,” he says. “This adds an extra layer of security.”
The study was a follow-up to the Symbiotic Human Robot Collaborative Assembly project, which was completed in 2019.