A wind power forecast is an estimate of the expected output of one or more wind turbines (known as a wind farm) in the near future. Production is frequently used to refer to the amount of available power for a wind farm (with units kW or MW depending on the wind farm’s nominal capacity). Forecasts in terms of energy can also be expressed by integrating power production over each time interval.
To better predict offshore wind power, Rutgers researchers created a machine learning model that uses a physics-based simulator and real-world meteorological data. The findings were published in the journal Applied Energy.
Offshore wind is rapidly maturing as a major source of renewable energy around the world, with growth projected to increase by 13% over the next two decades and 15-fold by 2040 to become a $1 trillion industry, matching capital spending on gas- and coal-fired power generation. In the United States, for example, the states of New York and New Jersey recently awarded two offshore wind energy contracts to help them meet their renewable energy integration goals.
Researchers have developed a machine learning model using a physics-based simulator and real-world meteorological data to better predict offshore wind power.
“We’re entering a new era of the offshore wind energy revolution,” said senior author Ruo-Qian (Roger) Wang, an assistant professor in Rutgers University-New Brunswick’s Department of Civil and Environmental Engineering. “The key to sustaining this expansion is the development of reliable tools for assessing and forecasting offshore wind turbine performance in order to improve project planning and support operations and maintenance. The 2019 Hornsea offshore wind farm blackout in England, as well as the 2021 Texas power crisis, highlight the critical need for powerful models to estimate and predict the environmental uncertainty of wind power generation.”
The power curve, or the relationship that governs the conversion of weather variables experienced by a wind turbine into electric power, is widely used in the wind industry to estimate power output for planning and operation. However, current methods for estimating power curves have limitations, such as relying solely on wind speed and ignoring other environmental factors, as well as ignoring the complex marine environment in which offshore turbines operate.
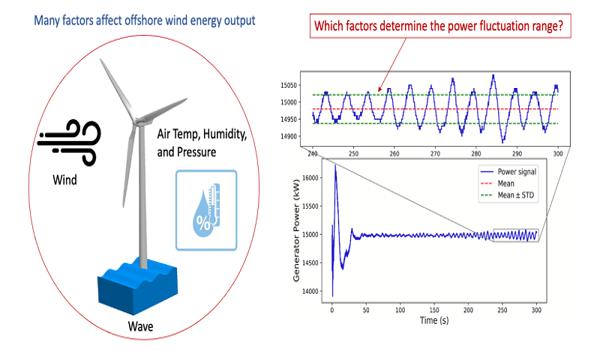
The Rutgers researchers created a sensitivity analysis framework in their study to identify and predict the major factors contributing to the environmental uncertainty of offshore wind power generation. A machine learning model drives this sensitivity analysis, fusing the outputs of a physics-based simulator with real-world meteorological data collected from a set of buoys deployed off the coast of New Jersey. The buoys are near at least three future offshore wind projects, which are expected to add about 2.8 gigatonnes of offshore wind capacity to the United States by 2024.
“To our knowledge, the proposed modeling framework is the first to investigate the impact of up to seven environmental variables, including wind- and wave-related factors, on offshore wind power generation,” said Aziz Ezzat, a co-author and assistant professor of Industrial and Systems Engineering at Rutgers. “The framework investigates the effect of variations in the offshore environment on the performance of the state-of-the-art 15-megawatt offshore turbine design, which is envisioned to be installed in the near future off the coasts of New Jersey and other U.S. states.”
The team’s analysis revealed that waves play a significant, if not the most significant, role in predicting the second moment of wind power, which is its variation around the mean generation level. The researchers also discovered that integrating several environmental variables can significantly improve the accuracy of predicting power output.
“When tested on real-world data from the New York and New Jersey sites, our analysis framework can improve accuracy by up to 91 percent over the traditional industrial standard for wind power estimation, which relies solely on wind speed,” Wang said. “Because of the significantly higher accuracy of our multi-input power estimation model, the research community and practitioners in the offshore wind industry should shift their focus to multi-input power estimation/prediction modeling tools, particularly in complex marine environments.”