Burn injuries are a common and serious problem, and accurately assessing the extent of a burn is crucial for determining the appropriate treatment. Traditionally, assessing burn injuries has involved invasive methods such as biopsy, which can be painful and time-consuming.
Researchers used a handheld scanner in conjunction with a neural network based on terahertz spectroscopy to predict burn healing with 93% accuracy. By guiding surgical treatment plans, the research has the potential to significantly improve burn healing outcomes.
Researchers created a neural network model that uses terahertz time-domain spectroscopy (THz-TDS) data to assess burns non-invasively. They combined the new method with a handheld imaging device designed specifically for fast THz-TDS imaging of burn injuries.
“It is critical for healthcare professionals to accurately assess the depth of a burn in order to provide the most appropriate treatment,” said M. Hassan Arbab of Stony Brook University. “Current methods of determining burn depth, which rely on visual and tactile examination, have been shown to be unreliable, with accuracy rates ranging from 60-75%. Our new method may improve the accuracy of burn severity assessments and aid in treatment planning.”
It is critical for healthcare professionals to accurately assess the depth of a burn in order to provide the most appropriate treatment. Current methods of determining burn depth, which rely on visual and tactile examination, have been shown to be unreliable, with accuracy rates ranging from 60-75%.
M. Hassan Arbab
M. Hassan Arbab
THz-TDS probes a sample with short pulses of terahertz radiation. It is being studied for assessing burn injuries because physical changes caused by a burn will cause changes in the terahertz reflectivity of the skin.
The researchers report their findings in the Optica Publishing Group journal Biomedical Optics Express, demonstrating that their artificial neural network classification algorithm can accurately predict the ultimate healing outcome of in vivo burns in an animal study with 93% accuracy. The new method reduces the amount of training data required by at least two orders of magnitude when compared to previous machine learning approaches used by the researchers. This could make processing large data sets obtained from large clinical trials more practical.
“In 2018, approximately 416,000 patients were treated for burn injuries in emergency departments in the United States alone,” said Arbab. “Our research has the potential to significantly improve burn healing outcomes by guiding surgical treatment plans, which could have a major impact on reducing the length of hospital stays and number of surgical procedures for skin grafting while also improving rehabilitation after injury.”
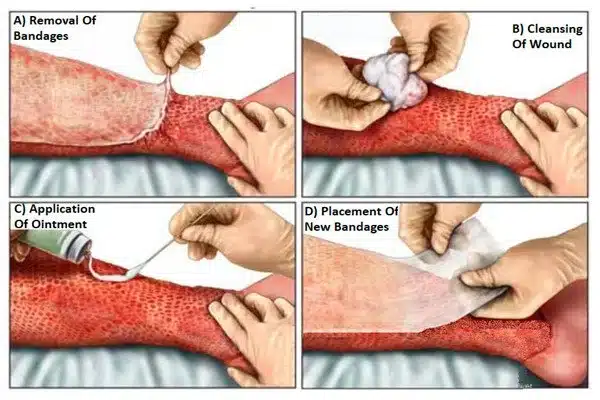
Better burn assessment
Various technologies to improve burn assessment have been developed, but they have not been widely adopted in the clinic due to drawbacks such as long acquisition times, high costs, and limited penetration depth and field of view. Although THz-TDS appears to be promising for burn assessment, initial demonstrations were limited to point spectroscopy measurements, which do not account for burn heterogeneity or spatial variations. THz spectroscopy setups are also bulky and expensive, requiring time-consuming optical alignments, making them unsuitable for clinical use in real-world settings.
“To address these challenges, we developed the portable handheld spectral reflection (PHASR) scanner, a user-friendly device for fast hyperspectral imaging of in vivo burn injuries using THz-TDS,” said Arbab. “This handheld device uses a dual-fiber-femtosecond laser with a center wavelength of 1560 nm and terahertz photoconductive antennas in a telecentric imaging configuration for the rapid imaging of a 37 x 27 mm2 field of view in just a few seconds.”
Previously, the researchers used numerical methods to extract features from THz-TDS images, as well as machine learning techniques to estimate the severity grade of in vivo burn injuries using PHASR scanner measurements. This method, however, did not take into account the physical dynamics and macroscopic changes in the dielectric permittivity of burned skin tissue. The response of a material to an electric field is described by dielectric permittivity.
To investigate the mechanisms that change the complex dielectric function of skin burns in terahertz frequencies, the researchers turned to the double Debye theory, which has been successfully used to explain the interaction of THz radiation with various types of biological tissue.
Predicting severity and healing
“We developed a neural network model utilizing the five parameters obtained from fitting the double Debye model to the dielectric permittivity of burn injuries,” said Arbab. “This physics-based approach allows for the extraction of biomedical diagnostic markers from broadband THz pulses, reducing the dimensionality of THz data for training the artificial intelligence models and improving the efficiency of machine learning algorithms.”
The researchers put their method to the test by taking spectroscopic images of skin burns and measuring their permittivity. Following the determination of the Debye parameters, the researchers used this information to build a neural network model based on labeled biopsies. The model predicted the outcome of the wound healing process with an accuracy rate of 93% and estimated the severity of the burns with an average accuracy rate of 84.5%.
The researchers emphasize that clinical testing of both the technique and the handheld imaging device is required before this technique can be integrated into the current clinical burn assessment workflow.