Baylor College of Medicine researchers built a machine learning system called SPLS-DA to seek for epigenetic markers for schizophrenia by studying certain sections of the human genome. By studying DNA from blood samples, the researchers discovered epigenetic markers that distinguished patients with schizophrenia from those who did not have the disorder. Using this data, the team developed a model that might utilize predictive analytics to estimate the likelihood of a person having schizophrenia.
According to a team lead by experts at Baylor College of Medicine, an unique technique that analyzes a section of the genome offers the possibility of early detection of schizophrenia. The technique used a machine learning algorithm known as SPLS-DA to study certain sections of the human genome known as CoRSIVs in the hopes of uncovering epigenetic markers for the disorder.
The scientists identified epigenetic markers, a profile of methyl chemical groups in DNA, that differ between those diagnosed with schizophrenia and people who do not have the disorder in DNA from blood samples and constructed a model that would estimate an individual’s likelihood of having the condition. Testing the model on an independent dataset demonstrated that it can correctly identify schizophrenia patients with an accuracy of 80%. The findings were published in the journal Translational Psychiatry.
Our study is new in a variety of ways,. We focused on CoRSIVs and employed the SPLS-DA machine learning technique to detect DNA methylation for the first time. Our findings are really fascinating to me as a scientist interested in using machine learning to medicine.
Dr. Chathura J. Gunasekara
“Schizophrenia is a severe condition that affects around 1% of the world’s population,” stated corresponding author Dr. Robert A. Waterland, a professor of pediatrics and nutrition at the USDA/ARS Children’s Nutrition Research Center at Baylor, as well as molecular and human genetics. “While genetic and environmental factors appear to be involved in the illness, existing research only explains a tiny number of instances, implying that additional factors, such as epigenetic, may also be important.”
Epigenetics is a mechanism for molecularly tagging DNA; it instructs different cells in the body which genes to switch on or off in that cell type; thus, epigenetic markers can vary between different normal tissues within the same individual. This makes determining whether epigenetic changes contribute to brain illnesses such as schizophrenia difficult.
To overcome this barrier, Waterland and his colleagues previously identified a collection of precise genomic locations in which DNA methylation, a common epigenetic marker, varies between persons but is constant across various organs in the same person. These genetic areas were dubbed CoRSIVs, short for correlated regions of systemic interindividual variation. They hypothesized that researching CoRSIVs is a fresh approach to discovering disease-causing epigenetic factors.
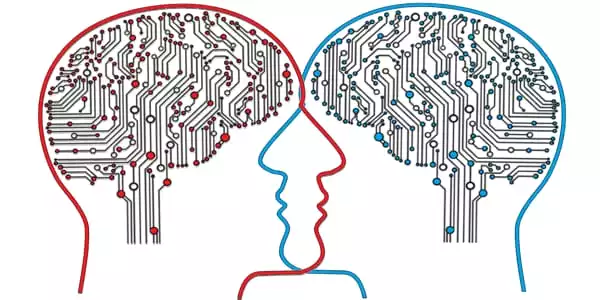
“Because methylation patterns in CoRSIVs are the identical in all organs of one individual,” Waterland explained, “we can evaluate them in a blood sample to infer epigenetic regulation on other regions of the body that are difficult to detect, such as the brain.”
According to the researchers, several prior studies have studied methylation profiles in blood samples with the purpose of detecting epigenetic variations between patients with schizophrenia.
“Our study is new in a variety of ways,” stated first author Dr. Chathura J. Gunasekara, a computer scientist at Waterland. “We focused on CoRSIVs and employed the SPLS-DA machine learning technique to detect DNA methylation for the first time. Our findings are really fascinating to me as a scientist interested in using machine learning to medicine. They not only offer the prospect of detecting schizophrenia risk early in life, but also outline a novel approach that might be applied to other disorders.”
The current study is particularly novel in that it considered important potential confounding factors that earlier studies did not consider. For example, smoking and taking antipsychotic medicines, both of which are common in schizophrenia patients, can have an impact on methylation patterns in the blood.
“We used a variety of methodologies to see if the methylation patterns we found at CoRSIVs were influenced by medicine or smoking. We were able to rule out that possibility” Waterland explained. “This, along with the fact that DNA methylation at CoRSIVs is formed very early in life, suggests that the epigenetic changes we discovered between schizophrenia patients and healthy persons existed before the disease was recognized, implying that they may contribute to the disorder.”
The researchers were able to create substantially stronger epigenetic markers associated with schizophrenia using this unique approach, according to the team. “We consider our study to be proof of principle that focusing on CoRSIVs allows for epigenetic epidemiology,” Waterland added.