Researchers can now access and analyze single-cell sequencing data thanks to the development of an algorithm that continuously processes new data. Researchers are still learning about the cells that make up our organs and are in charge of our health. Single-cell sequencing is a new technique that allows researchers to recognize and categorize cell types based on their characteristics. However, it generates a large amount of data, which a new algorithm may help to accelerate.
The fact that the human body is composed of cells is a fundamental and well-understood concept. Despite this, scientists are still attempting to identify the various types of cells that comprise our organs and contribute to our health.
Single-cell sequencing, a relatively new technique, allows researchers to recognize and categorize cell types based on characteristics such as which genes they express. This type of research, however, generates massive amounts of data, with datasets ranging from hundreds of thousands to millions of cells.
A new algorithm uses online learning to analyze large single-cell data sets using the amount of memory found on a standard laptop computer.
A new algorithm developed by Joshua Welch, Ph.D., of the Department of Computational Medicine and Bioinformatics, Ph.D. candidate Chao Gao, and their team employs online learning, greatly speeding up the process and allowing researchers all over the world to analyze large data sets using the amount of memory found on a standard laptop computer. The research was published in the journal Nature Biotechnology.
The method allows researchers to analyze millions of cells using the memory available on a standard computer. “Our method enables anyone with a computer to perform analyses at the scale of an entire organism,” Welch says. “That’s exactly where the field is heading.”
The team demonstrated their proof of principle by using data sets from the National Institutes of Health’s Brain Initiative, a project aimed at understanding the human brain by mapping every cell that involves investigative teams from across the country, including Welch’s lab.
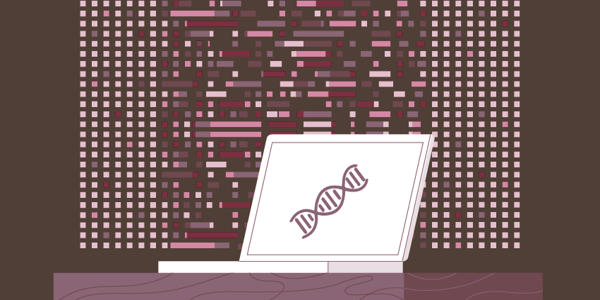
For projects like this one, each single-cell data set that is submitted must be re-analyzed with the previous data sets in the order they arrive, explains Welch. Their new approach allows new datasets to be added to existing ones without having to reprocess the older ones. It also allows researchers to divide datasets into so-called mini-batches in order to reduce the amount of memory required to process them.
“This is critical for the increasingly large sets containing millions of cells,” Welch says. “There have been five to six papers this year with two million cells or more, and the amount of memory required just to store the raw data is significantly more than anyone has on their computer.”
When applied to big data analytics tasks, traditional batch machine learning techniques have many limitations. A promising tool for data stream learning is an online learning technique with stream computing mode.
Welch compares the online technique to the continuous data processing performed by social media platforms such as Facebook and Twitter, which must process user-generated data on a continuous basis and serve up relevant posts to people’s feeds. “Instead of people tweeting, we have labs all over the world conducting experiments and releasing their data.”
The discovery has the potential to significantly improve efficiency for other large-scale projects such as the Human Body Map and the Human Cell Atlas. “Understanding the normal complement of cells in the body is the first step toward understanding how things go wrong in disease,” says Welch.