For computing, researchers constructed atomically thin artificial neurons capable of processing both light and electric information. The material allows different feedforward and feedback channels to exist within a neural network at the same time, increasing the capacity to tackle complicated issues.
Scientists have been researching how to replicate the flexible computing capabilities of biological neurons in order to produce faster and more energy-efficient machine learning systems for decades. One interesting technique is to employ memristors, which are electrical components that may store a value by altering their conductance and then use that value for in-memory processing.
However, combining both feedforward and feedback neural inputs has proven to be a significant barrier in duplicating the complicated operations of real neurons and brains using memristors. These systems, which rely on incentives and mistakes, support our cognitive ability to learn complicated tasks.
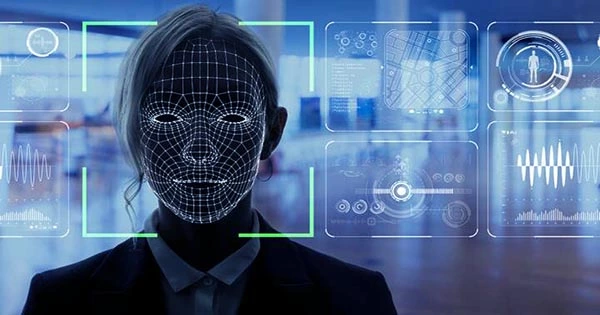
The construction of atomically thin artificial neurons built by stacking two-dimensional (2D) materials has been disclosed by a team of researchers from the University of Oxford, IBM Research Europe, and the University of Texas. The findings were reported in Nature Nanotechnology.
The researchers increased the capability of electronic memristors in the study by making them receptive to both optical and electrical inputs. This allowed the network to have distinct feedforward and feedback routes at the same time. The development enabled the researchers to develop winner-take-all neural networks, which are computational learning programs capable of addressing complicated machine learning issues such as unsupervised learning in clustering and combinatorial optimization problems.
2D materials are composed of only a few layers of atoms, and their tiny scale confers a variety of unique characteristics that may be fine-tuned depending on how the materials are stacked. The researchers created a device that changes its conductance based on the power and duration of light/electricity shone on it using a stack of three 2D materials—graphene, molybdenum disulfide, and tungsten disulfide—in this work.
These devices, unlike digital storage devices, are analog and function similarly to synapses and neurons in our human brain. The analog capability enables computations in which a series of electrical or optical signals received to the device results in slow changes in the quantity of stored electronic charge. This process serves as the foundation for neuronal computation threshold modes, which are akin to how our brain interprets a mix of excitatory and inhibitory impulses.
“This is a very exciting development,” stated lead author Dr. Ghazi Sarwat Syed, a Research Staff Member at IBM Research Europe Switzerland. Our research offered a revolutionary notion that outperforms the fixed feedforward operation often used in artificial neural networks today. Aside from the possible applications in AI hardware, these recent proof-of-concept discoveries show a major scientific development in the broader domains of neuromorphic engineering and algorithms, allowing us to better replicate and comprehend the brain.”
The experiment was carried out by Dr. Syed and Dr. Yingqiu Zhou (both DPhil students and lab colleagues at Oxford). According to Dr. Zhou, who is currently a Postdoctoral researcher at Denmark Technical University, their solution encapsulates the basic components of a biological neuron using low-dimensional optoelectronic physics.
They point out that the design of our heterostructure stack resulted in atomically abrupt semiconductor connections. The stack, in particular, provides a heterojunction that functions as the neural membrane, while the graphene electrodes that touch the heterojunction function as the neuronal soma. The neuronal state is therefore represented in the soma but changed by changes in the membrane, precisely as it is in genuine neurons.
As artificial intelligence applications have advanced at an exponential rate, the processing power required has overtaken the development of new hardware based on classical CPUs. There is an urgent need for innovative methodologies, such as the work of co-lead author Professor Harish Bhaskaran of the University of Oxford’s Advanced Nanoscale Engineering Laboratory and IBM Research Zurich. This process serves as the foundation for neuronal computation threshold modes, which are akin to how our brain interprets a mix of excitatory and inhibitory impulses.
“This is a very exciting development,” stated lead author Dr. Ghazi Sarwat Syed, a Research Staff Member at IBM Research Europe Switzerland. Our research offered a revolutionary notion that outperforms the fixed feedforward operation often used in artificial neural networks today. Aside from the possible applications in AI hardware, these recent proof-of-concept discoveries show a major scientific development in the broader domains of neuromorphic engineering and algorithms, allowing us to better replicate and comprehend the brain.”