The mechanism underlying the formation of emotional categories from visual facial expression data during development is largely unknown. As a result, from the standpoint of predictive processing theory, this study proposes a system-level explanation for understanding the facial emotion recognition process and its alteration in autism spectrum disorder (ASD). A hierarchical recurrent neural network was used to implement predictive processing for facial emotion recognition (RNN).
Face expressions are interpreted differently by people with an autism spectrum disorder. More information about how this occurs has been revealed by researchers. They created anomalies in a neural network model to investigate the effects on brain learning development. Autism spectrum disorder patients have difficulty interpreting facial expressions.
Tohoku University researchers have discovered how this happens by using a neural network model that simulates the brain on a computer. The findings were published in the journal Scientific Reports.
Artificial neural networks help researchers uncover new clues as to why people on the autism spectrum have trouble interpreting facial expressions.
“By observing facial expressions, humans can identify various emotions such as sadness and anger. However, little is known about how we learn to recognize different emotions based on visual cues from facial expressions “Yuta Takahashi, one of the paper’s co-authors, stated as much. “It’s also unclear what changes happen in this process that causes people with an autism spectrum disorder to struggle to read facial expressions.”
To learn more, the research team applied predictive processing theory. The brain, according to this theory, constantly predicts the next sensory stimulus and adapts when its prediction is incorrect. Sensory information, such as facial expressions, aids in prediction error reduction.
The predictive processing theory was incorporated into the artificial neural network model, which replicated the developmental process by learning to predict how parts of the face would move in videos of facial expression. Following that, the clusters of emotions self-organized into the higher level neuron space of the neural network model – despite the model not knowing which emotion the facial expression in the video corresponds to.
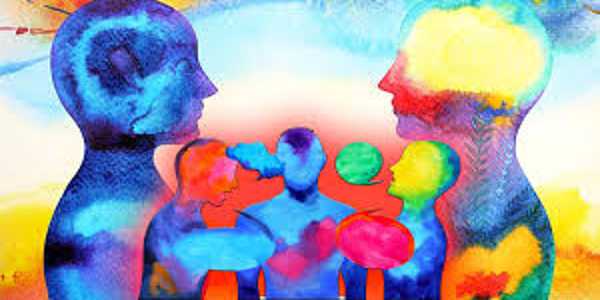
Forecasting methods based on simple mathematical models of the brain are known as artificial neural networks. They permit complex nonlinear relationships to exist between the response variable and its predictors. The model was able to generalize unknown facial expressions that were not taught to it, reproducing facial part movements and minimizing prediction errors.
Following that, the researchers carried out experiments and induced abnormalities in the activity of neurons to investigate the effects on learning development and cognitive characteristics. When the heterogeneity of activity in the neural population was reduced in the model, the ability to generalize was also reduced, and thus the formation of emotional clusters in higher-level neurons was inhibited. This resulted in an inability to identify the emotion of unknown facial expressions, a symptom similar to autism spectrum disorder.
According to Takahashi, the study demonstrated that predictive processing theory, when combined with a neural network model, can explain emotion recognition from facial expressions. “We hope to gain a better understanding of how humans learn to recognize emotions and the cognitive characteristics of people with autism spectrum disorder,” Takahashi added. “The research will aid in the development of appropriate intervention methods for people who have difficulty identifying emotions.”
A neural network is trained by varying the weights of neuron inputs based on the network’s performance on example inputs. When the network correctly classifies an image, the weights contributing to the correct answer are increased, while the weights contributing to the incorrect answer are decreased.
The network learns by examining individual records, generating a prediction for each record, and adjusting the weights whenever a prediction is incorrect. This process is repeated many times, and the network keeps improving its predictions until one or more of the stopping criteria are met.