It will be a long time before robots can prepare dinner, clear the kitchen table, and empty the dishwasher. First, robots must be able to distinguish the numerous things of various sizes, shapes, and brands in our houses. With a robotic system that uses artificial intelligence to assist robots better detect and remember objects, a team has made a big leap toward that technology.
In the Intelligent Robotics and Vision Lab at The University of Texas at Dallas, a robot slides a toy package of butter around a table. The robot is learning to recognize the object with each push, according to a novel method built by a team of UT Dallas computer scientists.
The new approach allows the robot to push things several times until a sequence of photos is recorded, at which point the system may segment all of the objects in the sequence until the robot recognizes them. Previously, methods relied on a single push or grip by the robot to “learn” the object.
The team presented its study paper at the Robotics: Science and Systems conference in Daegu, South Korea, from July 10 to 14. The conference papers are chosen based on their uniqueness, technical quality, significance, potential impact, and clarity.
After pushing the object, the robot learns to recognize it. With that data, we train the AI model so the next time the robot sees the object, it does not need to push it again. By the second time it sees the object, it will just pick it up.
Dr. Yu Xiang
It will be a long time before robots can prepare dinner, clear the kitchen table, and empty the dishwasher. However, the study team has made great progress with its robotic system, which uses artificial intelligence to assist robots in better identifying and remembering objects, according to Dr. Yu Xiang, senior author of the publication.
“If you ask a robot to pick up a mug or bring you a bottle of water, the robot needs to recognize those objects,” explained Xiang, an assistant professor of computer science at the Erik Jonsson School of Engineering and Computer Science.
The UTD researchers’ technology is designed to help robots detect a wide variety of objects found in environments such as homes and to generalize, or identify, similar versions of common items such as water bottles that come in varied brands, shapes or sizes.
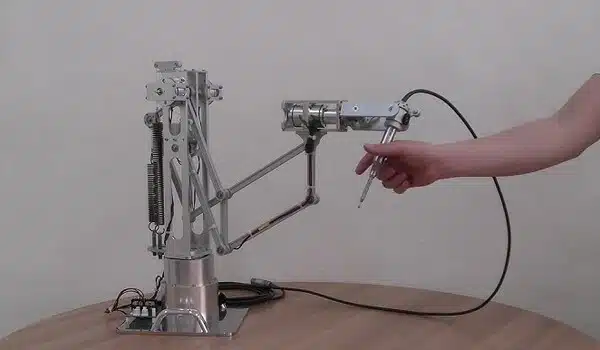
Inside Xiang’s lab is a storage bin full of toy packages of common foods, such as spaghetti, ketchup and carrots, which are used to train the lab robot, named Ramp. Ramp is a Fetch Robotics mobile manipulator robot that stands about 4 feet tall on a round mobile platform. Ramp has a long mechanical arm with seven joints. At the end is a square “hand” with two fingers to grasp objects.
Xiang said robots learn to recognize items in a comparable way to how children learn to interact with toys. “After pushing the object, the robot learns to recognize it,” Xiang said. “With that data, we train the AI model so the next time the robot sees the object, it does not need to push it again. By the second time it sees the object, it will just pick it up.”
The researchers’ method is unique in that the robot pushes each item 15 to 20 times, whereas earlier interactive perception methods only used a single push. Multiple pushes, according to Xiang, allow the robot to take additional photos with its RGB-D camera, which incorporates a depth sensor, to learn more about each item in greater detail. This decreases the possibility of errors.
One of the major functions required for robots to execute tasks is segmentation, which is the job of recognizing, discriminating, and remembering items. “To the best of our knowledge, this is the first system that leverages long-term robot interaction for object segmentation,” said Xiang.
Ninad Khargonkar, a PhD student in computer science, said working on the project has helped him develop the algorithm that assists the robot in making decisions. “It’s one thing to develop an algorithm and test it on an abstract data set; it’s quite another to test it out on real-world tasks,” Khargonkar explained. “Seeing that real-world performance – that was a key learning experience.”
The researchers’ next goal is to improve other functions, such as planning and control, which might enable jobs like sorting recycled materials.