Researchers develop explainable AI for decoding genome biology. Researchers at the Stowers Center for Medical Research, in cooperation with colleagues at Stanford University and the Technical University of Munich, have developed advanced explainable artificial intelligence (AI) in a technical tour de force to decode DNA encoded regulatory instructions. In a paper published online in Nature Genetics, the team showed that a neural network trained on high-resolution protein-DNA interaction maps will reveal subtle DNA sequence patterns throughout the genome and provide a deeper understanding of how these sequences are coordinated to control genes.
Neural networks are strong AI models that can learn complex patterns from a number of data types, such as images, speech signals, or text, to predict associated properties with amazing high precision. However, many believe these models to be uninterpretable since the learned predictive trends are difficult to derive from the model. This black-box existence hampered the widespread use of neural networks in biology, where the analysis of predictive patterns is of paramount importance.
Researchers found that a neural network trained on high-resolution maps of protein-DNA interactions can uncover subtle DNA sequence patterns throughout the genome and provide a deeper understanding of how these sequences are organized to regulate genes.
One of the main unanswered issues in biology is the second code of the genome—its regulatory code. The DNA bases (commonly represented by letters A, C, G, and T) encode not only instructions on how to produce proteins, but also when and where to make proteins in the body. The regulatory code is read by proteins called transcription factors that bind to a small strip of DNA called patterns. However, how precise combinations and pattern structures specify enforcement operation is an incredibly complicated question that has been difficult to pin down.
Now, an interdisciplinary team of biologists and computational researchers led by Stowers Investigator Julia Zeitlinger, PhD, and Anshul Kundaje, PhD, of Stanford University, have designed a neural network-named BPNet for Base Pair Network-that can be interpreted to reveal regulatory code by predicting transcription factor binding of DNA sequences with unparalleled precision. The key was to conduct transcription factor – DNA binding experiments and statistical modeling at the highest possible resolution, down to the level of individual DNA bases.
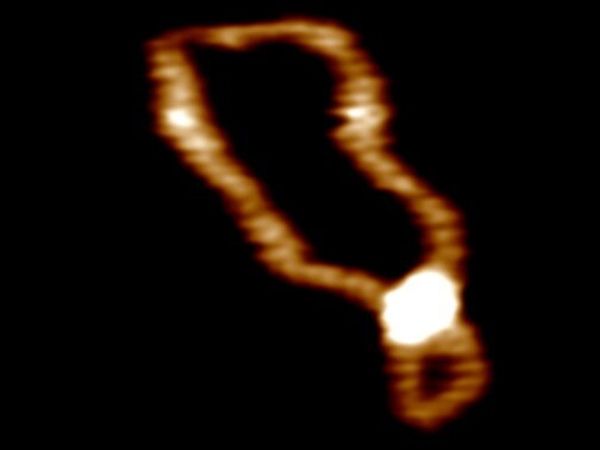
This increased resolution allowed them to create new interpretation tools to extract key elementary sequence patterns such as transcription factor binding patterns and combinatorial rules that combine patterns with regulatory code. “This was highly rewarding,” says Zeitlinger, “because the findings matched nicely with current experimental results, and also showed novel ideas that shocked us.”
For example, neural network models have helped researchers to discover a striking rule that regulates the binding of a well-studied transcription factor named Nanog. They find that Nanog cooperates with DNA when multiples of its motif occur regularly on the same side of the spiraling DNA helix.
“There has been a long trail of scientific evidence that this phenomenon of periodicity sometimes persists in the regulatory code,” Zeitlinger notes. “The precise circumstances is, however, elusive, and Nanog was not a suspect. It was shocking to learn that Nanog has such a pattern, and to see more descriptions of its interactions, since we did not specifically look for this pattern.”
“This is the main benefit of using neural networks to achieve this,” says Avsec, Ph.D., the first author of the article. Avsec and Kundaje created the first edition of the model when Avsec visited Stanford during his doctoral studies at Julien Gagneur’s lab, Ph.D., at the Technical University in Munich, Germany.
“More conventional bioinformatics methods model data using pre-defined rigid rules that are focused on known information. However, biology is incredibly rich and nuanced, “Well, says Avsec. “By using neural networks, we can train much more versatile and sophisticated models that learn complex patterns from scratch without prior experience, thereby facilitating new discoveries.”
The network architecture of BPNet is close to that of neural networks used for facial expression recognition. For example, the neural network first senses the edges in the pixels, then studies how the edges shape facial elements such as the eye, nose, or mouth, and eventually explores how the facial elements form a face together. Instead of learning from pixels, BPNet learns from the raw DNA sequence and learns how to identify sequence patterns and finally higher-order rules that predict base-resolution binding data.
Once the model has been conditioned to be very precise, the learned patterns are retrieved with interpreting software. The output signal is tracked back to the input sequence to show the patterns of the sequence. The final move is to use the model as an oracle and systematically consult it with complex DNA sequence designs, similar to what can be used to test theories experimentally, in order to discover the rules under which sequence patterns work in a combinatorial manner.
“The beauty is that the model can predict more sequence designs than we will test experimentally,” Zeitlinger says. “In addition, by forecasting the result of experimental disturbances, we can classify the most informative trials to test the model.” Indeed, with the aid of CRISPR gene editing tools, the researchers experimentally verified that the model’s predictions were very correct.
As the methodology is versatile and accessible to a number of various data types and cell types, it aims to contribute to an increasingly increasing understanding of the regulatory code and how genetic variation influences gene regulation. Both Zeitlinger Lab and Kundaje Lab are now using BPNet to accurately define binding patterns for other cell types, link patterns to biophysical parameters, and learn other structural features of the genome, such as those associated with DNA packaging. In order to encourage other scientists to use BPNet to tailor it to their own needs, researchers have made the entire program system accessible with documentation and tutorials.