According to a new method developed by a University of Alaska Fairbanks scientist, computers can be trained to detect distant nuclear detonations, chemical blasts, and volcano eruptions by learning from artificial explosion signals. The study was led by UAF Geophysical Institute postdoctoral researcher Alex Witsil and was recently published in the journal Geophysical Research Letters.
Witsil and colleagues at the Geophysical Institute’s Wilson Alaska Technical Center developed a library of synthetic infrared explosion signals to train computers to recognize the source of an infrared signal. Infrasound has a frequency that is too low for humans to hear and travels farther than high-frequency audible waves.
“We used modeling software to generate 28,000 synthetic infrasound signals, which, though generated in a computer, could hypothetically be recorded by infrasound microphones deployed hundreds of kilometers from a large explosion,” Witsil said.
We used modeling software to generate 28,000 synthetic infrasound signals, which, though generated in a computer, could hypothetically be recorded by infrasound microphones deployed hundreds of kilometers from a large explosion.
Alex Witsil
The artificial signals reflect changes in atmospheric conditions, which can change the signal of an explosion regionally or globally as sound waves propagate. These changes can make determining the origin and type of an explosion from a great distance difficult.
Why not use real-world examples of explosion sounds instead of making your own? There aren’t enough real-world examples to train generalized machine-learning detection algorithms because explosions haven’t occurred in every location on the planet and the atmosphere is constantly changing.
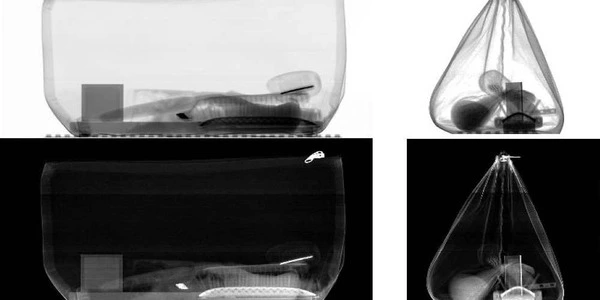
“We decided to use synthetics because we can model a number of different types of atmospheres through which signals can propagate,” Witsil said. “So even though we don’t have access to any explosions that happened in North Carolina, for example, I can use my computer to model North Carolina explosions and build a machine-learning algorithm to detect explosion signals there.”
Today, detection algorithms generally rely on infrasound arrays consisting of multiple microphones close to each other. For example, the international Comprehensive Test Ban Treaty Organization, which monitors nuclear explosions, has infrasound arrays deployed worldwide.
“That’s expensive, difficult to maintain, and there are a lot more things that can break,” Witsil explained. Witsil’s method enhances detection by utilizing hundreds of single-element infrared microphones that are already in use around the world. As a result, detection becomes more cost-effective.
The machine-learning method expands the utility of single-element infrared microphones by allowing them to detect more subtle explosion signals in near real-time. Single-element microphones are currently only useful for analyzing known and typically high-amplitude signals, as they did with the Tonga volcano’s massive eruption in January.
Witsil’s method could be used for national defense or natural disaster mitigation.