From “Law and Order” to “CSI,” not to mention in real life, investigators have relied on fingerprints as the gold standard for linking perpetrators to a crime. However, if a criminal leaf prints from separate fingers at two different crime locations, linking the scenes is difficult, and the trail may go cold.
It is widely acknowledged in the forensics community that fingerprints from different fingers of the same person—”intra-person fingerprints”—are distinct and thus unmatchable.
A team led by Columbia Engineering undergraduate senior Gabe Guo questioned this generally known assumption. Guo, who has no prior experience with forensics, discovered a public US government database of over 60,000 fingerprints and fed them in pairs into an artificial intelligence-based system known as a deep contrastive network. The pairings could belong to the same individual (but with different fingers) or to separate people.
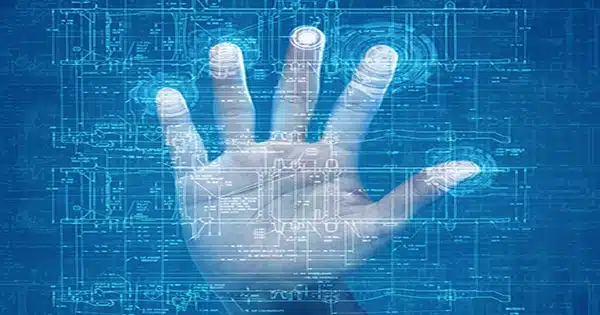
Over time, the AI system, which the team created by tweaking a cutting-edge framework, improved its ability to distinguish between supposedly identical fingerprints belonging to the same person and those that did not. The accuracy for a single pair was 77%. When numerous pairs were shown, accuracy improved dramatically, potentially enhancing current forensic efficiency by more than tenfold.
The project, a partnership between Hod Lipson’s Creative Machines lab at Columbia Engineering and Wenyao Xu’s Embedded Sensors and Computing lab at the University of Buffalo (SUNY), was published today in Science Advances.
Study findings challenge and amaze the forensics community.
After verifying its findings, the team promptly submitted them to a well-known forensics magazine, only to get a rejection a few months later. The anonymous professional reviewer and editor determined that “it is well known that every fingerprint is unique,” making it impossible to find similarities even if the fingerprints were from the same person.
The squad didn’t give up. They doubled down on the lead, feeding additional data into their AI system, and it continued to improve. Knowing the forensics community’s mistrust, the team chose to submit their book to a broader audience. The study was again denied, but Lipson, the James and Sally Scapa Professor of Innovation in the Department of Mechanical Engineering and co-director of the maker space Facility, filed an appeal.
“I don’t normally argue editorial decisions, but this finding was too important to ignore,” he told reporters. “If this information tips the balance, then I imagine that cold cases could be revived and even that innocent people could be acquitted.”
While the system’s accuracy is insufficient to make an official decision in a case, it can help rank leads in confusing scenarios. After much back and forth, the work was eventually accepted for publication by Science Advances.
A new type of forensic marker that accurately captures fingerprints
One of the sticky points was the following: What alternative information did the AI use to elude decades of forensic analysis? After carefully visualizing the AI system’s decision-making process, the researchers discovered that it was utilizing a new forensic marker.
“The AI was not using minutiae,’ which are the branchings and terminals in fingerprint ridges—the patterns used in traditional fingerprinting comparison,” said Guo, who started the research as a first-year student at Columbia Engineering in 2021. “Instead, it was using something else, related to the angles and curvatures of the swirls and loops in the center of the fingerprint.”
Aniv Ray, a Columbia Engineering senior, and Judah Goldfeder, a student who assisted with the data analysis, stated that their findings are only the beginning. “Just imagine how well this will perform once it’s trained on millions instead of thousands of fingerprints,” Ray went on to say.
The staff is aware that the data may be biased. The authors provide evidence that the AI performs similarly across genders and races where samples are available. They do, however, point out that if this technique is to be implemented in reality, more thorough validation with datasets with greater coverage is required.
Artificial intelligence can transform a well-established field.
This discovery is an example of many surprise things that will come from AI, notes Lipson. “Many people think that AI cannot make new discoveries-that it just regurgitates knowledge,” he went on to say. “But this research is an example of how even a fairly simple AI, given a fairly plain dataset that the research community has had lying around for years, can provide insights that have eluded experts for decades.”
He went on to say, “What’s even more exciting is that an undergraduate student with no background in forensics can successfully use AI to challenge a widely held belief in an entire field.” We are going to witness an explosion of AI-driven scientific discovery by non-experts, and the expert community, including academics, must prepare.”