The availability of fast and accurate simulation methods is critical in the research, development, and production of novel materials. Researchers will soon be able to develop complex material systems in a purely virtual environment thanks to machine learning, in which artificial intelligence (AI) autonomously acquires and applies new knowledge. What is the mechanism behind this, and which applications will benefit from it? A researcher from Karlsruhe Institute of Technology (KIT) and his colleagues from Göttingen and Toronto explain everything in an article published in the journal Nature Materials.
Digitization and virtualization are becoming more important in a variety of scientific disciplines. Materials science is one of these disciplines: the research, development, and production of novel materials rely heavily on the availability of fast and accurate simulation methods. This, in turn, benefits a wide range of different applications, ranging from efficient energy storage systems, such as those required for the use of renewable energies, to new medicines, the development of which requires an understanding of complex biological processes. AI and machine learning methods have the potential to advance simulations in the material sciences.
Research and development of novel materials depend heavily on the availability of fast and at the same time accurate simulation methods. Machine learning, in which AL autonomously acquires and applies new knowledge, will soon enable researchers to develop complex material systems in a purely virtual environment.
Sandia National Laboratories researchers have successfully used machine learning — computer algorithms that improve themselves by learning patterns in data — to complete time-consuming materials science calculations 40,000 times faster than usual. Their findings, which were published in the journal npj Computational Materials, could herald a dramatic acceleration in the development of new technologies for optics, aerospace, energy storage, and possibly medicine, while also saving laboratories money on computing costs.
“When compared to traditional simulation methods based on classical or quantum mechanical calculations, the use of neural networks specifically tailored to material simulations allows us to achieve a significant speed advantage,” explains physicist and AI expert Professor Pascal Friederich, Head of the AiMat — Artificial Intelligence for Materials Sciences research group at KIT’s Institute of Theorems and Probabilities (ITI). “With faster simulation systems, scientists will be able to develop larger and more complex material systems in a purely virtual environment, as well as comprehend and optimize them down to the atomic level.”
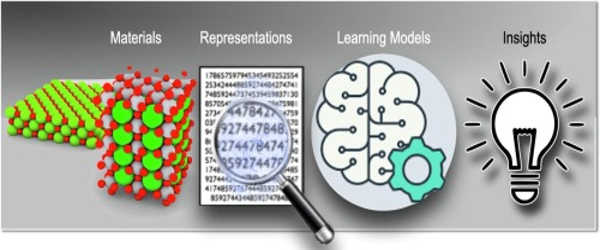
High Precision from the Atom to the Material
Pascal Friederich, associate group leader of the Nanomaterials by Information-Guided Design division at KIT’s Institute of Nanotechnology (INT), presents an overview of the basic principles of machine learning used for simulations in material sciences in an article published in Nature Materials with researchers from the University of Göttingen and the University of Toronto. This includes the data collection process as well as active learning methods. Machine learning algorithms allow artificial intelligence to not only process input data, but also to discover patterns and correlations in large data sets, learn from them, and make autonomous predictions and decisions.
It is critical for simulations in materials science to achieve high accuracy over a wide range of time and size scales, from the atom to the material, while keeping computational costs to a minimum. The scientists also discuss current applications, such as small organic molecules and large biomolecules, structurally disordered solid, liquid, and gaseous materials, and complex crystalline systems, such as metal-organic frameworks that can be used for gas storage or separation, sensors, or catalysts, in their article.
Even More Speed with Hybrid Methods
The researchers from Karlsruhe, Göttingen, and Toronto propose developing hybrid methods that combine machine learning (ML) and molecular mechanics (MM) methods in the future to further expand the possibilities of material simulations. MM simulations employ so-called force fields to calculate the forces acting on each individual particle and, as a result, predict motion. Because the ML and MM methods’ potentials are so similar, tight integration with variable transition areas is possible. In the future, these hybrid methods could significantly accelerate the simulation of large biomolecules or enzymatic reactions, for example.
Previously, machine learning was used to speed up simulations that calculate how atoms and molecules interact over time. The published results are the first to demonstrate the use of machine learning to accelerate simulations of materials at relatively large, microscopic scales, which the team anticipates will be of greater practical value to scientists and engineers.