Researchers used artificial intelligence to search through a vast chemical space of over a million molecules and optimize for several properties in order to find the right battery molecules. Scientists are looking for high-energy, stable batteries for the electric grid.
Adding new renewable energy sources such as wind and solar power to the electric grid will necessitate the use of specially designed large batteries that can charge during the day and provide energy at night. The flow battery is one type of battery that appears to be particularly promising for this purpose. Flow batteries are made up of two tanks of electrically active chemicals that exchange charge and can hold a lot of energy.
Researchers working on flow batteries are primarily concerned with identifying target molecules that can both store a large amount of energy and remain stable over long periods of time.
We’re essentially looking for needles in haystacks. When our model discovers something resembling a needle, it teaches itself how to find more. We are working on flow batteries are primarily concerned with identifying target molecules that can both store a large amount of energy and remain stable over long periods of time.
Hieu Doan
Researchers at the U.S. Department of Energy’s (DOE) Argonne National Laboratory used artificial intelligence (AI) to search through a vast chemical space of over a million molecules to find the right flow battery molecules. Finding the right molecules necessitates balancing several different characteristics. “We know that a majority of the molecules that we need in these batteries will have to satisfy multiple properties,” said Argonne chemist Rajeev Assary. “By optimizing multiple properties at the same time, we have a better chance of finding the best possible chemistry for our battery.”
Assary and his colleagues at Argonne’s Joint Center for Energy Storage Research modeled anolyte redoxmers, or electrically active molecules in a flow battery, in a new study that builds on work done last year. The researchers identified three properties for each redoxmer that they wanted to optimize. The first two, reduction potential and solvation free energy, are related to the amount of energy that the molecule can store. The third, fluorescence, acts as a self-reporting marker that indicates the battery’s overall health.
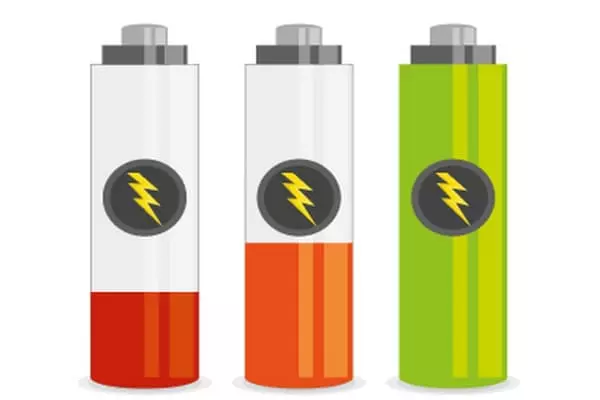
Because calculating the properties of interest for all potential candidates is extremely time consuming, the researchers turned to a machine learning and AI technique known as active learning, in which a model can actually train itself to identify increasingly plausible targets. “We’re essentially looking for needles in haystacks,” said Hieu Doan, an Argonne postdoctoral researcher. “When our model discovers something resembling a needle, it teaches itself how to find more.”
The researchers began with a relatively small “haystack” of 1400 redoxmer candidates whose properties they already knew from quantum mechanical simulations in order to make the most efficient use of active learning. They were able to see that the algorithm correctly identified the molecules with the best properties by using this dataset as practice.
“In previous research, we demonstrated how we could optimize one property at a time, but attempting to optimize several at once is a different type of challenge, and one that is probably more valuable for real-world conditions,” Assary explained. “Nature is never perfect, and no single molecule is in every way perfect. Our model enables us to experiment with various parameters in order to find the best fit.”
After exhausting the 1400-candidate set, the researchers broadened their search to a chemical space with a million different candidates. Better and better molecules were identified as the model’s performance improved iteratively. “We were encouraged by the fact that after only 100 molecules, our model was already finding molecules with more appealing properties than those in our original dataset,” Doan said.
According to Assary, the optimization algorithm could have applications other than flow batteries. He speculated that this algorithm could be applied to other types of batteries as well as other fields. “The mathematical approach we’re using is also widely used by stock traders and data scientists, demonstrating how common optimization problems are,” he explained.