Control software developed by biophysicists optimizes how fluorescence microscopes collect data on living samples. Their control loop, which was used to image mitochondrial and bacterial division sites in great detail, has been released as an open source plug-in and could inspire a new generation of intelligent microscopes.
Assume you’re a PhD student with a fluorescent microscope and a live bacteria sample. How should these resources be used to obtain detailed observations of bacterial division from the sample?
When bacterial division finally begins, you may be tempted to forego food and rest in order to sit at the microscope nonstop and acquire images. Manual detection and acquisition control is common in many sciences, so it’s not as crazy as it sounds.
Alternatively, you may want to set the microscope to take images indiscriminately and as often as possible. But excessive light depletes the fluorescence from the sample faster and can prematurely destroy living samples. Plus, you’d generate many uninteresting images, since only a few would contain images of dividing bacteria.
An intelligent microscope is kind of like a self-driving car. It needs to process certain types of information, subtle patterns that it then responds to by changing its behavior. By using a neural network, we can detect much more subtle events and use them to drive changes in acquisition speed.
Suliana Manley
Another approach would be to use artificial intelligence to detect precursors to bacterial division and use these to automatically update the microscope’s control software to capture more images of the event.
Yes, EPFL biophysicists have discovered a way to automate microscope control for imaging biological events in detail while limiting sample stress, all with the help of artificial neural networks. Their technique works for both bacterial cell division and mitochondrial division. Nature Methods describes the specifics of their intelligent microscope.
“An intelligent microscope is kind of like a self-driving car. It needs to process certain types of information, subtle patterns that it then responds to by changing its behavior,” explains principal investigator Suliana Manley of EPFL’s Laboratory of Experimental Biophysics. “By using a neural network, we can detect much more subtle events and use them to drive changes in acquisition speed.”
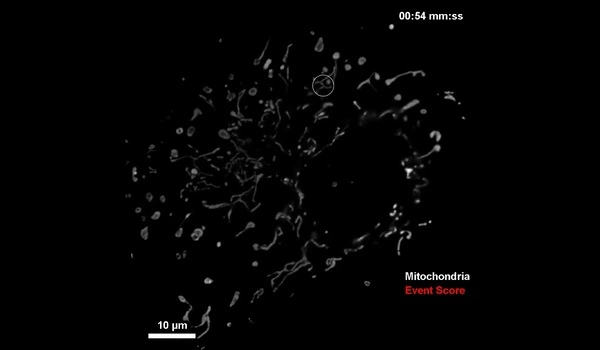
Manley and her colleagues first solved how to detect mitochondrial division, more difficult than for bacteria such as C. crescentus. Mitochondrial division is unpredictable, since it occurs infrequently, and can happen almost anywhere within the mitochondrial network at any moment. But the scientists solved the problem by training the neural network to look out for mitochondrial constrictions, a change in shape of mitochondria that leads to division, combined with observations of a protein known to be enriched at sites of division.
When both constrictions and protein levels are high, the microscope switches to high-speed imaging to capture a large number of detailed images of division events. To avoid exposing the sample to too much light, the microscope switches to low-speed imaging when constriction and protein levels are low.
The scientists demonstrated that using this intelligent fluorescent microscope, they could observe the sample for longer periods of time than standard fast imaging. Despite the fact that the sample was more stressed than in standard slow imaging, they were able to obtain more meaningful data.
“The potential of intelligent microscopy includes measuring what conventional acquisitions would miss,” explains Manley. “We can track each division in greater detail because we capture more events, measure smaller constrictions, and measure smaller constrictions.”
The control framework will be made available as an open source plug-in for the open microscope software Micro-Manager, allowing other scientists to integrate artificial intelligence into their own microscopes.