Scientists have developed and tested a computer-based artificial intelligence (AI) method to forecast the probability of early death due to chronic diseases in the middle ages. Researchers also shown that a computer algorithm developed using heart echocardiogram videos can estimate mortality within a year. The algorithm – an example of what is known as machine learning or artificial intelligence (AI) – outperformed other clinically used predictors, including pooled cohort equations and the Seattle Heart Failure score.
Researchers at Geisinger also discovered that a computer algorithm developed using heart echocardiogram videos can estimate mortality within a year.
Researchers develop a new machine-learning algorithm in which a video of echocardiograms is able to accurately predict patients who will die within a year. They have tested an artificial intelligence (AI)-based computer system to predict the risk of early death due to chronic diseases in a large middle-aged.
The algorithm – an example of what is known as machine learning, or artificial intelligence (AI) – outperformed other clinically used predictors, including pooled cohort equations and the Seattle Heart Failure score. The findings of the research have been published in Nature Biomedical Engineering.
“We were delighted to discover that machine learning can use unstructured datasets such as medical images and videos to improve a wide variety of clinical prediction models,” said Chris Haggerty, Ph.D., co-author and assistant professor at Geisinger’s Department of Translational Data Science and Informatics.
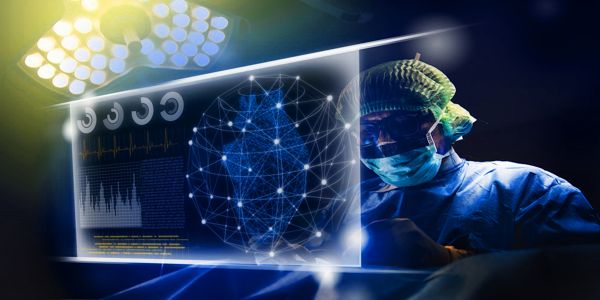
The AI machine learning models used in the current research are known as ‘random forest’ and ‘deep learning.’ This were associated with the conventional ‘Cox regression’ prediction model based on age and gender-found to be the least reliable for predicting mortality-and also with a multivariate Cox model that performed better but appeared to over-predict risk.
Imaging is essential to care outcomes in most medical areas and has become one of the most data-rich aspects of the electronic health record (EHR). For example, a single cardiac ultrasound yields approximately 3,000 photographs, and cardiologists have little time to view these images in the light of various other diagnostic results. This provides a major opportunity to exploit technologies, such as machine learning, handle and interpret this data, and eventually provide physicians with smart computer assistance.
Researchers have said that they have progressed the field of AI with their recent mortality prediction analysis. “We have taken a big step forward in this area by creating a novel and holistic approach to forecasting a person’s likelihood of premature death by machine learning,” said Dr. Stephen Weng, Assistant Professor of Epidemiology and Computer Science at the University of Nottingham, United Kingdom.
The study team used advanced computer hardware to train the machine learning algorithm in 812,278 echocardiogram videos obtained from 34,362 Geisinger patients over the last 10 years. The research compared the findings of the model to the predictions of cardiologists based on several surveys. A follow-up survey found that the accuracy of the cardiologist’s forecast increased by 13 per cent when supported by the model. Using nearly 50 million images, this analysis represents one of the largest medical imaging datasets ever released.
“Our aim is to create computational algorithms to optimize patient care,” said Alvaro Ulloa Cerna, PhD, author and senior data scientist at Geisinger’s Department of Translational Data Science and Informatics. “In this situation, we are delighted that our algorithm has been able to help cardiologists enhance their patient forecasts, since care and operation decisions are focused on these kinds of clinical predictions.”
The thesis was aligned with the already most influential field of research—diagnostic and prognosis—which has seen significant development in the usage of ML. Traditionally, forecasting has focused on statistics to estimate, for example, a person’s potential probability of contracting heart disease. These also shown high predictive accuracy, confirmed and repeated with multiple replication studies. “The challenge for applications and algorithms developed using ML is therefore not only to improve what can be done with conventional approaches, but also to build and report them in an equally straightforward and replicable manner,” the authors wrote.