Artificial intelligence (AI) can be used to detect Alzheimer’s disease from routine brain imaging tests such as MRI scans. AI algorithms can analyze these scans and detect subtle changes in brain structure and function that may indicate the presence of Alzheimer’s disease. There are various AI approaches being developed for this purpose, including deep learning algorithms that are trained on large datasets of brain scans and use neural networks to identify patterns and features associated with Alzheimer’s disease.
A deep learning-based method for detecting Alzheimer’s disease based on routinely collected clinical brain images has been developed and validated by researchers. Although researchers have made significant progress in detecting Alzheimer’s disease symptoms using high-quality brain imaging tests collected as part of research studies, a team at Massachusetts General Hospital (MGH) recently developed an accurate method for detection that relies on routinely collected clinical brain images. The advancement could lead to more precise diagnoses.
For the study, which is published in PLOS ONE, Matthew Leming, Ph.D., a research fellow at MGH’s Center for Systems Biology and an investigator at the Massachusetts Alzheimer’s Disease Research Center, and his colleagues used deep learning – a type of machine learning and artificial intelligence that uses large amounts of data and complex algorithms to train models.
Because Alzheimer’s disease typically affects older adults, deep learning models frequently struggle to detect the rarer early-onset cases. To address this, we made the deep learning model ‘blind’ to brain features that it found to be overly associated with the patient’s listed age.
Matthew Leming
In this case, the researchers created a model for detecting Alzheimer’s disease using data from brain magnetic resonance images (MRIs) collected from patients with and without Alzheimer’s disease who were seen at MGH prior to 2019.
The team then tested the model on five datasets, including MGH post-2019, Brigham and Women’s Hospital pre- and post-2019, and outside systems pre- and post-2019, to see if it could detect Alzheimer’s disease based on real-world clinical data, regardless of hospital or time.
The study included 11,103 images from 2,348 patients at risk for Alzheimer’s disease and 26,892 images from 8,456 patients who did not have the disease. The model detected Alzheimer’s disease risk with 90.2% accuracy across all five datasets.
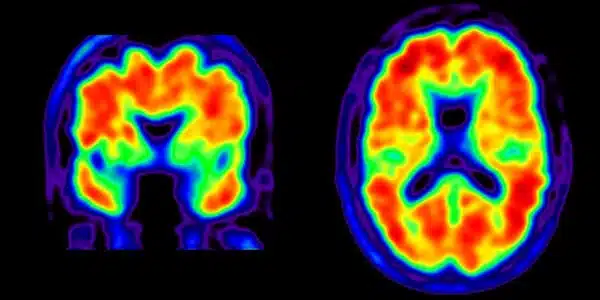
The ability to detect Alzheimer’s disease regardless of other variables such as age was one of the work’s main innovations. “Because Alzheimer’s disease typically affects older adults, deep learning models frequently struggle to detect the rarer early-onset cases,” explains Leming. “To address this, we made the deep learning model ‘blind’ to brain features that it found to be overly associated with the patient’s listed age.”
Another common challenge in disease detection, particularly in real-world settings, according to Leming, is dealing with data that is very different from the training set. A deep learning model trained on MRIs from a General Electric scanner, for example, may fail to recognize MRIs collected on a Siemens scanner.
The model used an uncertainty metric to determine whether the patient data were too different from what it had been trained on for it to make a successful prediction.
“This is one of the few studies that used routinely collected brain MRIs to try to detect dementia. While many deep learning studies for Alzheimer’s detection from brain MRIs have been conducted, this study took significant steps toward actually performing this in real-world clinical settings rather than perfect laboratory settings “said Leming. “Our findings, with cross-site, cross-time, and cross-population generalizability, make a strong case for clinical use of this diagnostic technology.”