KAUST researchers have laid the groundwork for more efficient hardware-based artificial intelligence computing systems by developing a biomimicking “spiking” neural network on a microchip.
Artificial intelligence technology is rapidly evolving, with a flood of new applications in advanced automation, data mining and interpretation, healthcare, and marketing, to name a few. These systems are built around a mathematical artificial neural network (ANN), which is made up of layers of decision-making nodes. Labeled data is first fed into the system to “train” the model to respond in a specific way, after which the decision-making rules are locked in and the model is deployed on standard computing hardware.
A neural network that mimics the biology of the brain can be loaded onto a microchip for faster and more efficient artificial intelligence.
While this method works, it is a clumsy approximation of our brains’ far more complex, powerful, and efficient neural networks. “An ANN is an abstract mathematical model that bears little resemblance to real nervous systems and necessitates a lot of computing power,” explains Wenzhe Guo, a Ph.D. student on the research team. “In contrast, a spiking neural network is built and operates in the same way as the biological nervous system, and it can process information faster and more efficiently.”
Spiking neural networks (SNNs) mimic the structure of the nervous system as a network of synapses that transmit information in the form of action potentials, or spikes, via ion channels as they occur. This event-driven behavior, which is mathematically implemented as a “leaky integrate-and-fire model,” makes SNNs extremely energy efficient. Furthermore, the structure of interconnected nodes allows for a high degree of parallelization, which increases processing power and efficiency. It is also amenable to direct implementation in computing hardware as a neuromorphic chip.
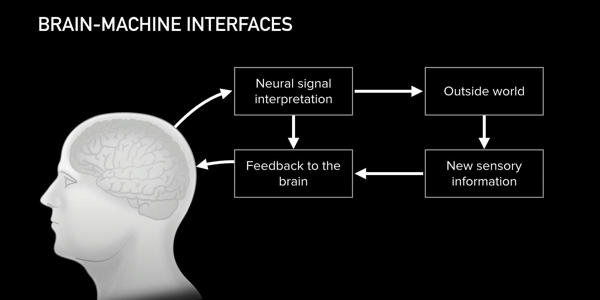
Pharmaceutical companies are spending more and more money on clinical trials while releasing fewer and fewer new therapies. Now is the time to find a way to speed up research. Testing on cell cultures in Petri dishes is insufficient to demonstrate the efficacy of a new therapy because cells do not react as they would in the human body when they are removed from their natural environment.
Guo explains, “We used a standard low-cost FPGA microchip and implemented a spike-timing-dependent plasticity model, which is a biological learning rule discovered in our brain.”
Importantly, no teaching signals or labels are required for this biological model, allowing the neuromorphic computing system to learn real-world data patterns without training. “Because SNN models are very complex,” Guo explains, “our main challenge was to tailor the neural network settings for optimal performance.” “We then designed the optimal hardware architecture while keeping cost, speed, and energy consumption in mind.”
The brain-on-a-chip developed by the team was found to be more than 20 times faster and 200 times more energy efficient than other neural network platforms. “Our ultimate goal is to create a brain-like hardware computing system that is compact, fast, and low-energy. The next step is to collaborate to improve the design and optimize product packaging, miniaturize the chip, and customize it for various industrial applications “Guo explains.
The migration of neural progenitors in the central nervous system’s complex tissue environment is poorly understood. Progress in this area has the potential to accelerate breakthroughs in neuroregenerative therapies, brain cancer treatments, and neurodevelopmental research.