The researchers created a machine learning-based model to predict the properties of bonded systems. They were able to predict the binding energy, bond length, number of covalent electrons, and Fermi energy using the density of states of the individual component reactants. The broadly applicable model is expected to contribute significantly to the development of materials such as catalysts and nanowires.
Researchers working in fields ranging from catalysis to solar cells face a challenge in designing materials with the necessary properties to perform specific functions. Modeling approaches can be used to predict information and guide refinements to speed up development processes. Researchers from The University of Tokyo Institute of Industrial Science created a machine learning model to predict the properties of bonded and adsorbed materials based on individual component parameters. Their findings have been published in the journal Applied Physics Express.
The length and strength of bonds in materials, for example, play critical roles in determining the structures and properties we observe on the macroscopic scale. The ability to easily predict these properties is thus useful when designing new materials.
Researchers have developed a machine learning-based model to predict the characteristics of bonded systems. Using the density of states of the individual component reactants, they have achieved accurate predictions of the binding energy, bond length, number of covalent electrons, etc.
Individual atoms, molecules, and materials can all have their density of states (DOS) calculated. Simply put, it describes the possibilities for electrons that arrange themselves in a material. An appealing tool is a modeling approach that can take this information for selected components and produce useful data for the desired product – without the need to make and analyze the material.
The researchers used a machine learning approach to predict four different properties of products from the DOS information of the individual components. Although the DOS has previously been used as a descriptor to establish single parameters, this is the first time that multiple properties have been predicted.
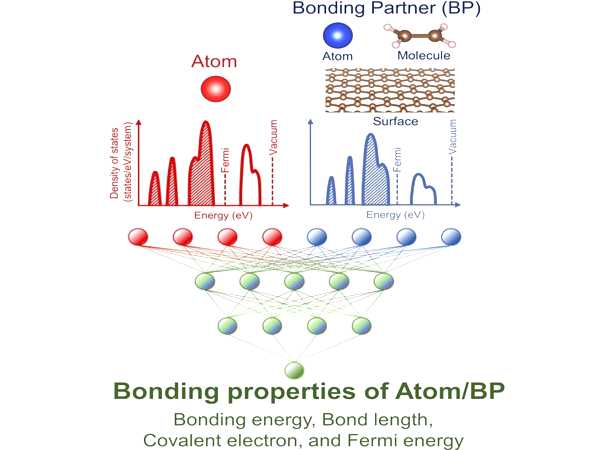
Researchers from The University of Tokyo Institute of Industrial Science report a machine learning-based model for predicting the bonding properties of materials. “We were able to quantitatively predict the binding energy, bond length, number of covalent electrons, and Fermi energy after bonding for three different general types of systems,” says Eiki Suzuki, the study’s first author. “And our predictions were very accurate across the board.”
The analysis is relatively efficient because the calculation of DOS in an isolated state is less complex than in bonded systems. Furthermore, even when only 20% of the dataset was used for training, the neural network model performed well.
Bonding properties such as length and strength are critical for material structure and properties. In this case, a machine learning (ML) model is used to predict bonding properties from information about isolated systems prior to bonding. The density of states (DOS) before the bond formation is used as the ML descriptor in this model, and it accurately predicts the binding energy, bond distance, covalent electron amount, and Fermi energy even when only 20% of the entire dataset is used for training.
“One significant advantage of our model is that it is general and can be applied to a wide variety of systems,” study co-author Teruyasu Mizoguchi says. “We believe that our findings could have a significant impact on numerous development processes, such as catalysis, and could be especially useful in newer research areas such as nanoclusters and nanowires.”