A team of TUM researchers has created a new early warning system for vehicles that uses artificial intelligence to learn from thousands of real-world traffic situations. The system was studied in collaboration with the BMW Group. The results show that, when used in today’s self-driving cars, it can warn seven seconds ahead of potentially critical situations that the cars cannot handle on their own – with over 85 percent accuracy.
To make self-driving cars safer in the future, development efforts frequently rely on sophisticated models designed to give cars the ability to analyze the behavior of all traffic participants. But what if the models aren’t yet capable of dealing with complex or unexpected situations?
In general, self-driving cars are expected to make road traffic safer by removing the factor of “human error.” However, until such a vehicle is capable of handling all situations completely autonomously, it must be able to recognize and process all possible situations. Existing systems quickly reach their limits because some situations are difficult to predict or simply too complex.
Researchers have developed a new early warning system for vehicles that uses artificial intelligence to learn from thousands of real traffic situations.
A team led by Prof. Eckehard Steinbach, Chair of Media Technology and member of the Board of Directors of TUM’s Munich School of Robotics and Machine Intelligence (MSRM), is taking a novel approach. Their system can learn from previous situations in which self-driving test vehicles were pushed to their limits in real-world road traffic, thanks to artificial intelligence (AI). These are situations in which a human driver takes over, either because the car indicates a need for intervention or because the driver decides to intervene for safety reasons.
During road tests, the researchers identified approximately 2,500 situations that required driver intervention; they discovered that the system issued warnings about potentially critical situations seven seconds in advance with greater than 85 percent accuracy. The system recognizes patterns in data collected by sensors and cameras using a recurrent neural network and will warn drivers if it detects a situation that the control system has had difficulty handling in the past.
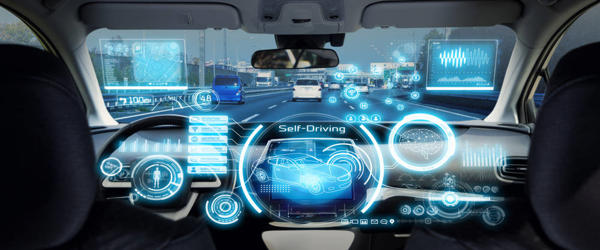
Pattern recognition through RNN
Sensors and cameras are used to capture surrounding conditions and record vehicle status data such as steering wheel angle, road conditions, weather, visibility, and speed. Based on the data, the AI system, which is based on a recurrent neural network (RNN), learns to recognize patterns. If the system detects a pattern in a new driving situation that the control system was unable to handle previously, the driver will be warned of a potentially dangerous situation.
“Many existing methods for making vehicles more autonomous study what the cars currently understand about traffic and then attempt to improve the models that they use. The main advantage of our technology is that we completely disregard what the car thinks. Instead, we confine ourselves to data based on what actually occurs and search for patterns “according to Steinbach “As a result, the AI uncovers potentially critical situations that models may be incapable of recognizing or have yet to discover. As a result, our system includes a safety function that detects when and where the cars have flaws.”
Warnings up to seven seconds in advance
The researchers tested the technology on public roads with the BMW Group and its autonomous development vehicles, analyzing approximately 2500 situations in which the driver had to intervene. According to the research, AI is already capable of predicting potentially critical situations with greater than 85% accuracy – up to seven seconds before they occur.
Collecting data with no extra effort
Large amounts of data are required for the technology to function. After all, AI can only recognize and predict experiences at the system’s limits if the situations have been seen before. With so many development vehicles on the road, the data practically generated itself, according to Christopher Kuhn, one of the study’s authors: “Every time a potentially critical situation arises on a test drive, we end up with a new training example.” Because the data is stored centrally, each vehicle can learn from all of the data collected across the entire fleet.