In a study conducted by the Ottawa Faculty of Medicine, an AI-based deep-learning model 93 a birth defect with % accuracy. It improves AI’s ability to serve as an aid in the quick and accurate reading of ultrasound images. Dr. Mark Walker and his colleagues at the University of Ottawa conducted an experiment to demonstrate the use of deep-learning architecture in the early and reliable diagnosis of cystic hygroma.
Cystic hygroma is a congenital defect in which the lymphatic vascular system develops abnormally. It is a rare disorder that causes swelling around the head and neck as a result of fluid accumulation and can be fatal. Though the condition can be easily detected in the prenatal stage with an ultrasound, the researchers wished to gauge how well AI-driven pattern recognition could do the job. And the results have been nothing short of spectacular.
Researchers at the University of Ottawa’s Faculty of Medicine are pioneering the use of a unique Artificial Intelligence-based deep learning model as an assistive tool for the rapid and accurate reading of ultrasound images in a new proof-of-concept study led by Dr. Mark Walker.
What we demonstrated was that in the field of ultrasound, we can use the same tools for image classification and identification with high sensitivity and specificity.
Dr. Walker
The study’s goal was to show that deep-learning architecture has the potential to support early and reliable identification of cystic hygroma from first trimester ultrasound scans. Cystic hygroma is an embryonic condition that causes an abnormal development of the lymphatic vascular system. It is a rare and potentially fatal disorder characterized by fluid swelling around the head and neck.
Dr. Walker, co-founder of The Ottawa Hospital’s OMNI Research Group (Obstetrics, Maternal and Newborn Investigations), and his research group wanted to see how well AI-driven pattern recognition could detect the birth defect during an ultrasound appointment.
The researchers used a dataset of nearly 300 fetal ultrasounds collected retrospectively at Ottawa Hospital. The images were correctly identified as cases of cystic hygroma compared to normal controls using a DenseNet model. This was accomplished by calculating specificity, sensitivity, and other metrics. To evaluate model interpretability, gradient class activation heat maps were created, which visualize pixels in images. The overall accuracy of the model was 93%.
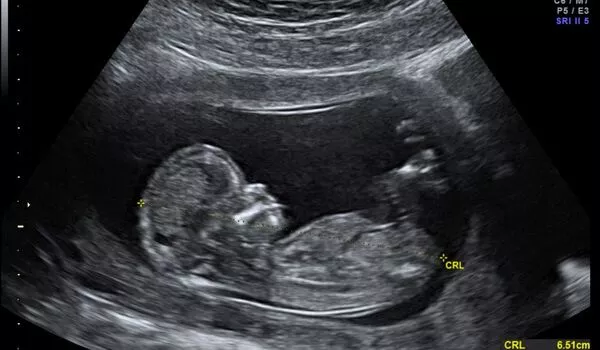
“What we demonstrated was that in the field of ultrasound, we can use the same tools for image classification and identification with high sensitivity and specificity,” says Dr. Walker, who believes their approach could be applied to other fetal anomalies commonly identified by ultrasound.
The study’s goal was to show that deep-learning architecture has the potential to support early and reliable identification of cystic hygroma from first trimester ultrasound scans. The researchers wanted to show how deep-learning architecture can help with the early and reliable detection of cystic hygroma from first-trimester ultrasound scans. Cystic hygroma is an embryonic condition that causes abnormal development of the lymphatic vascular system.
The Ottawa-led research group believes that this study could lead to several advancements in the future. With further development, the team believes their approach might be applied to other fetal anomalies generally identified by ultrasonography, including testing in a large multi-site dataset.
According to Dr. Walker, the group’s goal is to form an international consortium to upload obstetrical ultrasound images to the cloud, which is a network of remote servers that stores data and provides computing services to other users. This could eventually assist physicians in developing countries in achieving cloud-based interpretation and diagnosis.
Walker hopes to form an international consortium in the future to upload ultrasound images to remote servers, allowing physicians in low and middle-income countries to make more accurate diagnoses. This necessitates larger datasets from multiple sources being fed to the AI.