Neural networks are used in artificial intelligence to conduct calculations digitally using microelectronic chip technology. Leipzig University physicists have developed a neural network that operates on active colloidal particles rather than electricity. The researchers detail how these microparticles might be utilized as a physical system for artificial intelligence and time series prediction in a paper published in Nature Communications.
“Our neural network belongs to the field of physical reservoir computing, which uses the dynamics of physical processes, such as water surfaces, bacteria, or octopus tentacle models, to make calculations,” says Professor Frank Cichos, whose research group developed the network with ScaDS.AI support. Since 2019, the research facility with campuses in Leipzig and Dresden has been sponsored as part of the German government’s AI Strategy, with assistance from the Federal Ministry of Education and Research and the Free State of Saxony.
Our neural network belongs to the field of physical reservoir computing, which uses the dynamics of physical processes, such as water surfaces, bacteria, or octopus tentacle models, to make calculations.
Professor Frank Cichos
“In our realization, we use synthetic self-propelled particles that are only a few micrometres in size,” explains Cichos. “We show that these can be used for calculations and at the same time present a method that suppresses the influence of disruptive effects, such as noise, in the movement of the colloidal particles.” Colloidal particles are particles that are finely dispersed in their dispersion medium (solid, gas or liquid).
For their experiments, the physicists developed tiny units made of plastic and gold nanoparticles, in which one particle rotates around another, driven by a laser. These units have certain physical properties that make them interesting for reservoir computing. “Each of these units can process information, and many units make up the so-called reservoir. We change the rotational motion of the particles in the reservoir using an input signal. The resulting rotation contains the outcome of a calculation,” explains Dr Xiangzun Wang. “Like many neural networks, the system needs to be trained to perform a particular calculation.”
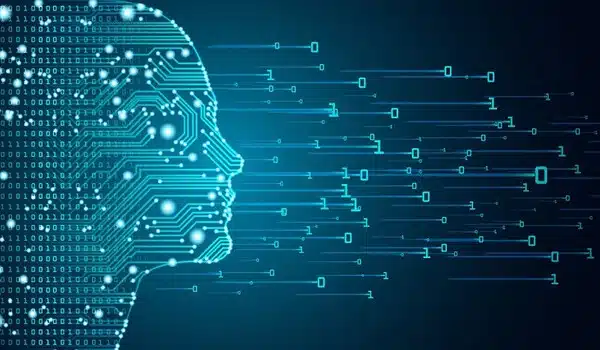
Active microparticles refer to tiny particles that can exhibit dynamic behaviors or responses to external stimuli. These particles have garnered interest across various fields, including materials science, medicine, and electronics.
The researchers were especially interested in noise. “Because our system contains extremely small particles in water, the reservoir is subject to strong noise, similar to the noise that all molecules in a brain are subject to,” Professor Cichos said.
“This noise, Brownian motion, adversely disturbs the reservoir computer’s operation and often necessitates a very large reservoir to rectify. In our research, we discovered that leveraging previous reservoir states can increase computer performance, allowing smaller reservoirs to be employed for specific computations under noisy situations.”
Cichos adds that this has not only advanced the subject of information processing using active matter, but has also resulted in a strategy for optimizing reservoir calculation by minimizing noise.