There are many different types of software that use artificial intelligence (AI) to help interpret complex data. These include machine learning algorithms, natural language processing (NLP) systems, and computer vision systems, among others. These technologies can be used for a wide range of applications, such as image and speech recognition, language translation, and predictive modeling. Additionally, some software can also help to extract insights from data, identify patterns and trends, and make predictions.
Experiment data is frequently not only multidimensional but also noisy and riddled with artifacts. This makes interpreting the data difficult. Now, a team at HZB has created software that uses self-learning neural networks to intelligently compress data and reconstruct a low-noise version in the next step. This enables the identification of correlations that would otherwise be undetectable. The software has now been used successfully in photon diagnostics at DESY’s FLASH-free electron laser. However, it is applicable to a wide range of scientific applications.
More is not always better, but it can also be a problem. Correlations are frequently lost when dealing with highly complex data that has many dimensions due to the numerous parameters. Especially since experimentally obtained data are additionally disturbed and noisy due to influences that cannot be controlled.
We were able to extract this information from noisy electron time-of-flight data much better than with traditional analysis methods.
Dr. Gregor Hartmann
Helping humans to interpret the data
Now, new software based on artificial intelligence methods can help: It is a special class of neural networks (NN) that experts call “disentangled variational autoencoder network (β-VAE).” Put simply, the first NN takes care of compressing the data, while the second NN subsequently reconstructs the data. “In the process, the two NNs are trained so that the compressed form can be interpreted by humans,” explains Dr. Gregor Hartmann. The physicist and data scientist supervises the Joint Lab on Artificial Intelligence Methods at HZB, which is run by HZB together with the University of Kassel.
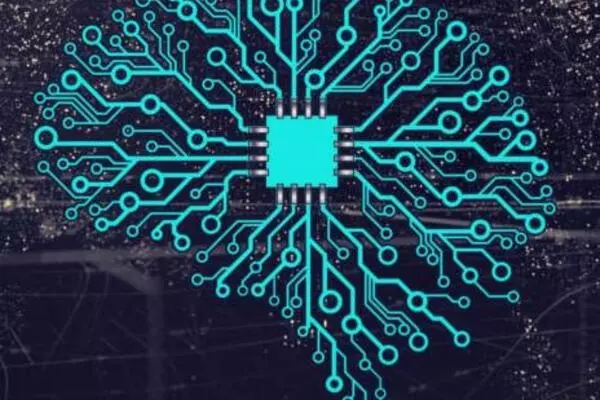
Extracting core principles without prior knowledge
Google Deepmind had already proposed to use β-VAEs in 2017. Many experts assumed that the application in the real world would be challenging, as non-linear components are difficult to disentangle. “After several years of learning how the NNs learn, it finally worked,” says Hartmann. β-VAEs are able to extract the underlying core principle from data without prior knowledge.
Photon energy of FLASH determined
The software was used to calculate the photon energy of FLASH from single-shot photoelectron spectra in the newly published study. “We were able to extract this information from noisy electron time-of-flight data much better than with traditional analysis methods,” Hartmann says. This method can be used to clean up data that contains detector-specific artifacts.
A powerful tool for different problems
“When it comes to impaired data, the method is really good,” Hartmann emphasizes. The program can even reconstruct tiny signals that are not visible in raw data. In large experimental data sets, such networks can aid in the discovery of unexpected physical effects or correlations. “AI-based intelligent data compression is a very powerful tool, not just in photon science,” Hartmann explains.
Now plug and play
Hartmann and his team spent three years developing the software in total. “However, it is now more or less plug and play. We hope that many colleagues will come with their data soon so that we can assist them.”