Autonomous vehicles have the potential to alleviate traffic congestion, improve traffic flow through vehicle-to-vehicle communication, and revolutionize the travel experience by providing comfortable and safe rides. Furthermore, incorporating autonomous driving technology into electric vehicles may contribute to more environmentally friendly transportation solutions.
The ability of autonomous vehicles to detect and navigate around obstacles, pedestrians, and other vehicles in a variety of environments is a critical requirement for their success. Smart sensors such as LiDARs (Light Detection and Ranging) for a 3D view of the surroundings and depth information, RADaR (Radio Detection and Ranging) for detecting objects at night and in cloudy weather, and a set of cameras for providing RGB images and a 360-degree view are used in current autonomous vehicles, forming a comprehensive dataset known as point cloud. These sensors, however, frequently face challenges such as reduced detection capabilities in adverse weather, on unstructured roads, or due to occlusion.
Our proposed system operates in real-time, enhancing the object detection capabilities of autonomous vehicles, and making navigation through traffic smoother and safer.
Prof. Jeon
To overcome these shortcomings, an international team of researchers led by Professor Gwanggil Jeon from the Department of Embedded Systems Engineering at Incheon National University (INU), Korea, has recently developed a groundbreaking Internet-of-Things-enabled deep learning-based end-to-end 3D object detection system. “Our proposed system operates in real-time, enhancing the object detection capabilities of autonomous vehicles, making navigation through traffic smoother and safer,” explains Prof. Jeon. Their paper was made available online on October 17, 2022, and published in Volume 24, Issue 11 of the journal IEEE Transactions on Intelligent Transport Systems on November 2023.
The proposed innovative system is based on the deep learning object detection technique YOLOv3 (You Only Look Once), which is the most active state-of-the-art technique available for 2D visual detection. The researchers used this new model to detect 2D objects first, then modified the YOLOv3 technique to detect 3D objects. The system generates bounding boxes with confidence scores and labels for visible obstacles as output using both point cloud data and RGB images as input.
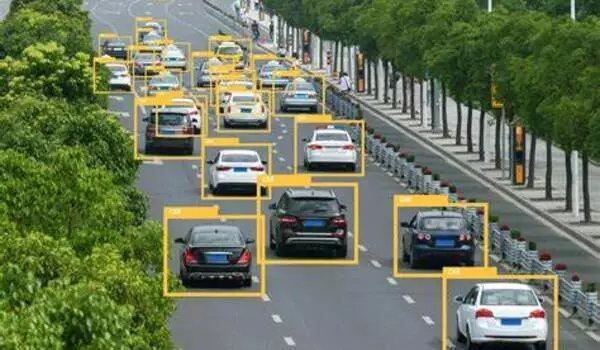
The team tested the system’s performance using the Lyft dataset, which consisted of road data captured from 20 autonomous vehicles traveling a predetermined route in Palo Alto, California, over a four-month period. The results showed that YOLOv3 outperforms other cutting-edge architectures in terms of accuracy. Specifically, the overall accuracy for 2D and 3D object detection was 96% and 97%, respectively.
Prof. Jeon emphasizes the potential impact of this enhanced detection capability: “By improving detection capabilities, this system could propel autonomous vehicles into the mainstream. The introduction of autonomous vehicles has the potential to transform the transportation and logistics industry, offering economic benefits through reduced dependence on human drivers and the introduction of more efficient transportation methods.”
Furthermore, the current work is expected to stimulate research and development in a variety of technological fields, including sensors, robotics, and artificial intelligence. Recognizing the current emphasis on 2D image development, the team intends to investigate additional deep-learning algorithms for 3D object detection in the future.
In summary, this groundbreaking study could pave the way for the widespread adoption of self-driving cars, resulting in a more environmentally friendly and comfortable mode of transportation.